素晴らしいllms-datasets
- トレーニング前のコーパス、微調整命令データセット、優先データセット、評価データセット、および従来のNLPデータセットの5つの次元にわたって既存のLLMSテキストデータセットを要約します。 (定期的な更新)
- 新しいデータセットセクションが追加されています。マルチモーダル大手言語モデル(MLLMS)データセット、検索拡張生成(RAG)データセット。 (段階的な更新)
紙
論文「大規模な言語モデルのデータセット:包括的な調査」がリリースされました。
抽象的な:
このペーパーは、LLMSの顕著な進歩に重要な役割を果たす大規模な言語モデル(LLM)データセットへの探査に乗り出します。データセットは、LLMの開発を維持および育成するルートシステムに類似した基礎インフラストラクチャとして機能します。その結果、これらのデータセットの調査は、研究の重要なトピックとして現れます。 LLMデータセットの包括的な概要と徹底的な分析の現在の欠如に対処し、現在の状況と将来の傾向に関する洞察を得るために、この調査はLLMデータセットの基本的な側面を5つの視点から統合および分類します。トレーニングコーパス; (2)指示微調整データセット。 (3)優先データセット。 (4)評価データセット。 (5)従来の自然言語処理(NLP)データセット。この調査は、一般的な課題に光を当て、将来の調査のための潜在的な道を指摘しています。さらに、444のデータセットからの統計、8つの言語カテゴリをカバーし、32のドメインにまたがる既存のデータセットリソースの包括的なレビューも提供されています。 20次元からの情報は、データセット統計に組み込まれています。調査された総データサイズは、トレーニング前のコーパスの場合は774.5 TBを上回り、他のデータセットでは700mインスタンスを上回ります。 LLMテキストデータセットの景観全体を提示し、この分野の研究者向けの包括的なリファレンスとして機能し、将来の研究に貢献することを目指しています。
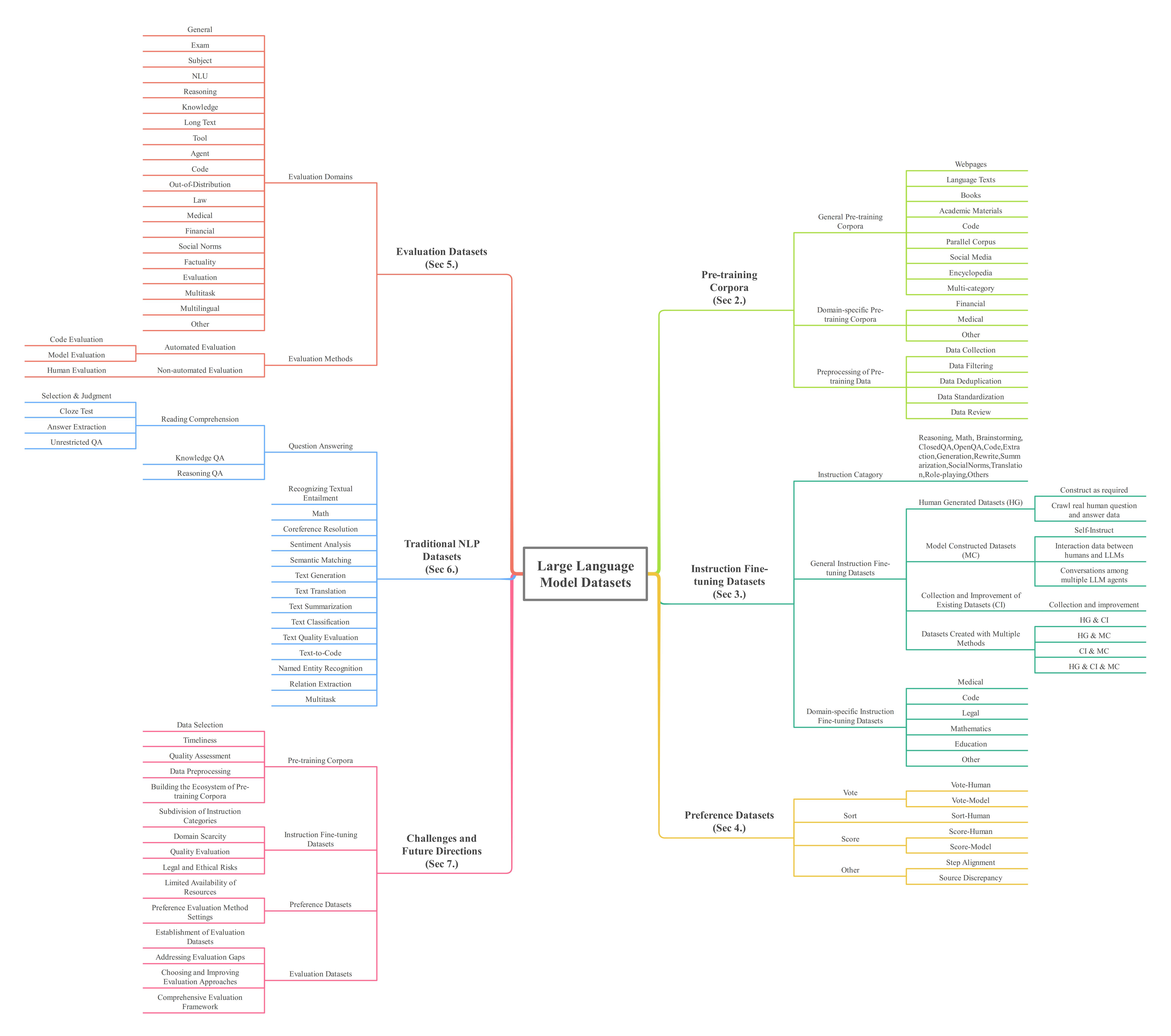
図1。調査の全体的なアーキテクチャ。より良いビューを得るためにズームインします
データセット情報モジュール
以下は、データセット情報モジュールの概要です。
- コーパス/データセット名
- 出版社
- リリース時間
- サイズ
- 公開かどうか
- 「すべて」は完全なオープンソースを示します。
- 「部分的」は、部分的にオープンソースを示します。
- 「NOT」はオープンソースではないことを示します。
- ライセンス
- 言語
- 「en」は英語を示します。
- 「Zh」は中国人を示しています。
- 「AR」はアラビア語を示しています。
- 「es」はスペイン語を示します。
- 「ru」はロシア語を示しています。
- 「de」はドイツ語を示します。
- 「KO」は韓国語を示しています。
- 「LT」はリトアニア人を示しています。
- 「FA」はペルシャ/ファルシを示しています。
- 「PL」はプログラミング言語を示します。
- 「Multi」は多言語を示し、括弧内の数は含まれる言語の数を示します。
- 建設方法
- 「HG」は、ヒト生成されたコーパス/データセットを示します。
- 「MC」は、モデル構築されたコーパス/データセットを示します。
- 「CI」は、既存のコーパス/データセットの収集と改善を示しています。
- カテゴリ
- ソース
- ドメイン
- 命令カテゴリ
- 優先評価方法
- 「vo」は投票を示します。
- 「だから」はソートを示します。
- 「SC」はスコアを示します。
- 「-H」は人間によって行われたことを示します。
- 「-m」は、モデルによって実施されることを示します。
- 質問タイプ
- 「SQ」は主観的な質問を示します。
- 「OQ」は客観的な質問を示します。
- 「Multi」は複数の質問タイプを示します。
- 評価方法
- 「CE」はコード評価を示します。
- 「彼」は人間の評価を示しています。
- 「私」はモデルの評価を示します。
- 集中
- 評価カテゴリ/サブカテゴリの数
- 評価カテゴリ
- エンティティカテゴリの数(NERタスク)
- 関係カテゴリの数(REタスク)
Changelog
- ( 2024/01/17
- (2024/02/02 Dolma (トレーニング前のコーパス|一般的なトレーニング以前のコーパス|マルチカテゴリ)を追加します。
- (2024/02/15 ayaコレクションを追加します(命令微調整データセット|一般的な命令微調整データセット| HG&CI&MC); AYAデータセット(命令微調整データセット|一般的な命令微調整データセット| HG)。
- (2024/02/22) OpenMathinstruct-1を追加します(命令微調整データセット|ドメイン固有の命令微調整データセット| Math); Finben (評価データセット|金融)。
- (2024/04/05)
- 新しいデータセットセクションの追加: (1)マルチモーダル大手言語モデル(MLLMS)データセット。 (2)検索拡張生成(RAG)データセット。
- MMRS-1Mを追加します(MLLMSデータセット|命令微調整データセット); videoChat2-IT (MLLMSデータセット|命令微調整データセット); InstructDoc (MLLMSデータセット|命令微調整データセット); allava-4vデータ(MLLMSデータセット|命令微調整データセット); MVBench (MLLMSデータセット|評価データセット); Olympiadbench (MLLMSデータセット|評価データセット); MMMU (MLLMSデータセット|評価データセット)。
- 手がかりベンチマークシリーズ(評価データセット|評価プラットフォーム)を追加します。 OpenllMリーダーボード(評価データセット|評価プラットフォーム); OpenCompass (評価データセット|評価プラットフォーム); MTEBリーダーボード(評価データセット|評価プラットフォーム); C-MTEBリーダーボード(評価データセット|評価プラットフォーム)。
- nah(heedle-in-a-haystack) (評価データセット|長いテキスト); Tooleyes (評価データセット|ツール); uhgeval (評価データセット|事実性); Clongeval (評価データセット|長いテキスト)。
- MathPileを追加(トレーニング前のコーパス|ドメイン固有のプリトレーニングコーパス|数学); wanjuan-cc (トレーニング前のコーパス|一般的なトレーニング以前のコーパージ|ウェブページ)。
- IEPILEを追加します(命令微調整データセット|一般命令微調整データセット| CI); Instantie (命令微調整データセット|一般的な命令微調整データセット| HG)。
- crud-rag (rag datasets)を追加します。 wikieval (ragデータセット); RGB (RAGデータセット); Rag-Instruct-Benchmark-Tester (Rag Datasets); ARES (RAGデータセット)。
- (2024/04/06)
- GPQA (評価データセット|件名)を追加します。 MGSM (評価データセット|多言語); Halueval-Wild (評価データセット|事実性); cmath (評価データセット|件名); Finemath (評価データセット|件名);リアルタイムQA (評価データセット|事実性); wyweb (評価データセット|件名);中国Facteval (評価データセット|事実性);カウントスター(評価データセット|長いテキスト)。
- Slimpajama (事前トレーニングコーパス|一般的なトレーニング以前のコーパス|マルチカテゴリ)を追加します。 MassiveText (トレーニング前のコーパス|一般的なトレーニング以前のコーパス|マルチカテゴリ); Madlad-400 (トレーニング前のコーパス|一般的なトレーニング前コーパージ|ウェブページ); Minerva (トレーニング前のコーパス|一般的なトレーニング以前のコーパス|マルチカテゴリ); ccaligned (トレーニング前のコーパス|一般的な訓練前コーパス); wikimatrix (事前トレーニングコーパス|一般的なトレーニング前コーパス|並列コーパス); OpenWebMath (トレーニング前のコーパス|ドメイン固有のプリトレーニングコーパス|数学)。
- WebQuestions (従来のNLPデータセット|質問応答|知識QA)を追加します。
- Alce (RAGデータセット)を追加します。
- アルファフィンを追加します(命令微調整データセット|ドメイン固有の命令微調整データセット|その他); COIG-CQIA (命令微調整データセット|一般的な命令微調整データセット| HG&CI)。
- (2024/06/15)
- 手がかりを追加(評価データセット|医療); CHCベンチ(評価データセット|一般); CIFベンチ(評価データセット|一般); aclue (評価データセット|件名); LESC (評価データセット| NLU); alignbench (評価データセット|マルチタスク); sciknoweval (評価データセット|件名)。
- MAP-CCを追加(トレーニング前のコーパス|一般的なトレーニング以前のコーパス|マルチカテゴリ); fineweb (トレーニング前のコーパス|一般的なトレーニング以前のコーパージ|ウェブページ); CCI 2.0 (トレーニング前のコーパス|一般的なトレーニング以前のコーパージ|ウェブページ)。
- WildChat (命令微調整データセット| MC)を追加します。
- OpenHermespReferences (設定データセット|ソート)を追加します。 huozi_rlhf_data (優先データセット|投票); helpSteer (優先データセット|スコア); helpSteer2 (優先データセット|スコア)。
- MMTベンチ(MLLMSデータセット|評価データセット)を追加します。モスカー(MLLMSデータセット|事前トレーニングコーパス); MM-Niah (MLLMSデータセット|評価データセット)。
- CRAG (RAGデータセット)を追加します。
- (2024/08/29)
- GameBench (評価データセット|推論)を追加します。 Halludial (評価データセット|事実性); WildBench (評価データセット|一般); domaineval (評価データセット|コード); Sysbench (評価データセット|一般); kobest (評価データセット| nlu); sarcasmbench (評価データセット| nlu); C 3ベンチ(評価データセット|件名);テーブルベンチ(評価データセット|推論); arablegaleval (評価データセット|法律)。
- MultiTrust (MLLMSデータセット|評価データセット)を追加します。 obelisc (MLLMSデータセット|トレーニング前のコーパス); Multimed (MLLMSデータセット|評価データセット)。
- DCLMを追加します(トレーニング前のコーパス|一般的なトレーニング以前のコーパージ| Webページ)。
- Lithuanian-QA-V1 (命令微調整データセット| CI&MC)を追加します。 Reintruct (命令微調整データセット| HG&CI&MC); kollmコンバージョン(命令微調整データセット| ci)。
- (2024/09/04)
- LongWriter-6K (命令微調整データセット| CI&MC)を追加します。
- medtrinity-25m (MLLMSデータセット|評価データセット)を追加します。 MMIU (MLLMSデータセット|評価データセット)。
- Expository-Prose-V1 (トレーニング前のコーパス|一般的なトレーニングコーポラ|マルチカテゴリ)を追加します。
- DebateQA (評価データセット|知識)を追加します。ニードルベンチ(評価データセット|ロングテキスト); ArabAcmmlu (評価データセット|件名); perianmmlu (評価データセット|件名); tmmlu+ (評価データセット|件名)。
- Rageval (Rag Datasets)を追加します。 LFRQA (RAGデータセット); MultiHop-Rag (RAGデータセット)。
- データセット情報をCSV形式でリリースします。
目次
- トレーニング前のコーパス
- 一般的なトレーニングのコーパス
- ウェブページ
- 言語テキスト
- 本
- アカデミック資料
- コード
- 平行コーパス
- ソーシャルメディア
- 百科事典
- マルチカテゴリ
- ドメイン固有のプリトレーニングコーパス
- 指示微調整データセット
- 一般的な命令微調整データセット
- 人間の生成データセット(HG)
- モデル構築されたデータセット(MC)
- 既存のデータセット(CI)の収集と改善
- HG&CI
- HG&MC
- CI&MC
- HG&CI&MC
- ドメイン固有の命令微調整データセット
- 優先データセット
- 評価データセット
- 一般的な
- テスト
- 主題
- NLU
- 推論
- 知識
- 長いテキスト
- 道具
- エージェント
- コード
- ood
- 法
- 医学
- 金融
- 社会的規範
- 事実
- 評価
- マルチタスク
- 多言語
- 他の
- 評価プラットフォーム
- 従来のNLPデータセット
- 質問に答える
- テキストの含意を認識します
- 数学
- コアレファレンス解像度
- 感情分析
- セマンティックマッチング
- テキスト生成
- テキスト翻訳
- テキストの要約
- テキスト分類
- テキスト品質評価
- テキストからコード
- 名前付きエンティティ認識
- 関係抽出
- マルチタスク
- マルチモーダル大手言語モデル(MLLMS)データセット
- トレーニング前のコーパス
- 指示微調整データセット
- 評価データセット
- 検索拡張生成(RAG)データセット
トレーニング前のコーパス
トレーニング前のコーパスは、LLMSのトレーニング前プロセスで使用されるテキストデータの大規模なコレクションです。
一般的なトレーニングのコーパス
一般的なトレーニングのコーパスは、多様なドメインとソースからの広範なテキストで構成される大規模なデータセットです。それらの主な特徴は、テキストコンテンツが単一のドメインに限定されておらず、一般的な基礎モデルのトレーニングにより適していることです。コーパスは、データカテゴリに基づいて分類されます。
データセット情報形式:
- Dataset name Release Time | Public or Not | Language | Construction Method | Paper | Github | Dataset | Website
- Publisher:
- Size:
- License:
- Source:
ウェブページ
CC-Stories 2018-6 | |ではありませんen | ci |論文| github |データセット
- 出版社:Google Brain
- サイズ:31 GB
- ライセンス: -
- 出典:Common Crawl
CC100 2020-7 |すべて| Multi(100)| ci |論文|データセット
- 出版社:Facebook AI
- サイズ:2.5 TB
- ライセンス:一般的なクロール条件
- 出典:Common Crawl
cluecorpus2020 2020-3 |すべて| Zh | ci |論文|データセット
- 出版社:手がかりの組織
- サイズ:100 GB
- ライセンス:MIT
- 出典:Common Crawl
一般的なクロール2007-X |すべて|マルチ| hg | Webサイト
- 出版社:Common Crawl
- サイズ: -
- ライセンス:一般的なクロール条件
- 出典:Web Crawlerデータ
Culturax 2023-9 |すべて| Multi(167)| ci |論文|データセット
- 出版社:オレゴン大学等。
- サイズ:27 TB
- ライセンス:MC4&OSCARライセンス
- 出典:MC4、オスカー
C4 2019-10 |すべて| en | ci |論文|データセット
- 出版社:Google Research
- サイズ:12.68 TB
- ライセンス:ODC-By&Common Crawlの使用条件
- 出典:Common Crawl
MC4 2021-6 |すべて| Multi(108)| ci |論文|データセット
- 出版社:Google Research
- サイズ:251 GB
- ライセンス:ODC-By&Common Crawlの使用条件
- 出典:Common Crawl
オスカー22.01 2022-1 |すべて| Multi(151)| ci |論文|データセット| Webサイト
- 出版社:INRIA
- サイズ:8.41 TB
- ライセンス:CC0
- 出典:Common Crawl
RealNews 2019-5 |すべて| en | ci |論文| github
- 出版社:ワシントン大学等。
- サイズ:120 GB
- ライセンス:Apache-2.0
- 出典:Common Crawl
redpajama-v2 2023-10 |すべて| Multi(5)| ci | github |データセット| Webサイト
- 出版社:一緒にコンピューター
- サイズ:30.4 Tトークン
- ライセンス:一般的なクロール条件
- 出典:Common Crawl、C4など。
RefinedWeb 2023-6 |部分的| en | ci |論文|データセット
- 出版社:ファルコンLLMチーム
- サイズ:5000 GB
- ライセンス:ODC-1.0
- 出典:Common Crawl
wudaocorpora-text 2021-6 |部分的| Zh | hg |論文|データセット
- 出版社:Baai et al。
- サイズ:200 gb
- ライセンス:MIT
- 出典:中国のウェブページ
wanjuan-cc 2024-2 |部分的| en | hg |論文|データセット
- 出版社:上海の職人インテリジェンス研究所
- サイズ:1トークン
- ライセンス:CC-By-4.0
- 出典:Common Crawl
Madlad-400 2023-9 |すべて| Multi(419)| hg |論文| github |データセット
- 出版社:Google Deepmind et al。
- サイズ:2.8 Tトークン
- ライセンス:ODL-by
- 出典:Common Crawl
FineWeb 2024-4 |すべて| en | ci |データセット
- 出版社:Huggingfacefw
- サイズ:15 TBトークン
- ライセンス:ODC-1.0
- 出典:Common Crawl
CCI 2.0 2024-4 |すべて| Zh | hg | DataSet1 | DataSet2
- 出版社:Baai
- サイズ:501 GB
- ライセンス:CCIの使用の悪化
- 出典:中国のウェブページ
DCLM 2024-6 |すべて| en | ci |論文| github |データセット| Webサイト
- 出版社:ワシントン大学等。
- サイズ:279.6 TB
- ライセンス:一般的なクロール条件
- 出典:Common Crawl
言語テキスト
ANC 2003-X |すべて| en | hg | Webサイト
- 出版社:米国科学財団ら。
- サイズ: -
- ライセンス: -
- 出典:American English Texts
BNC 1994-X |すべて| en | hg | Webサイト
- 出版社:オックスフォード大学出版局等。
- サイズ:4124テキスト
- ライセンス: -
- 出典:英国の英語のテキスト
News-Crawl 2019-1 |すべて| Multi(59)| hg |データセット
- 出版社:Ukri et al。
- サイズ:110 GB
- ライセンス:CC0
- 出典:新聞
本
アンナのアーカイブ2023-x |すべて|マルチ| hg | Webサイト
- 出版社:アンナ
- サイズ:586.3 TB
- ライセンス: -
- 出典:Sci-Hub、Library Genesis、Z-Libraryなど。
bookcorpusopen 2021-5 |すべて| en | ci |論文| github |データセット
- 出版社:ジャック・バンディ等。
- サイズ:17,868冊の本
- ライセンス:Smashwords利用規約
- 出典:トロントブックコーパス
PG-19 2019-11 |すべて| en | hg |論文| github |データセット
- 出版社:DeepMind
- サイズ:11.74 GB
- ライセンス:Apache-2.0
- 出典:Project Gutenberg
プロジェクトGutenberg 1971-X |すべて|マルチ| hg | Webサイト
- 出版社:Ibiblio et al。
- サイズ: -
- ライセンス:プロジェクトGutenberg
- 出典:電子ブックデータ
Smashwords 2008-x |すべて|マルチ| hg | Webサイト
- 出版社:Draft2Digital et al。
- サイズ: -
- ライセンス:Smashwords利用規約
- 出典:電子ブックデータ
トロントブックコーパス2015-6 | |ではありませんen | hg |論文| Webサイト
- 出版社:トロント大学等。
- サイズ:11,038冊の本
- ライセンス:MIT&Smashwordsの利用規約
- 出典:Smashwords
アカデミック資料
コード
BigQuery 2022-3 | |ではありませんpl | ci |論文| github
- 出版社:Salesforce Research
- サイズ:341.1 gb
- ライセンス:Apache-2.0
- 出典:BigQuery
Github 2008-4 |すべて| pl | hg | Webサイト
- 出版社:Microsoft
- サイズ: -
- ライセンス: -
- 出典:さまざまなコードプロジェクト
PHI-1 2023-6 | |ではありませんen&pl | HG&MC |論文|データセット
- 出版社:Microsoft Research
- サイズ:7 bトークン
- ライセンス:CC-BY-NC-SA-3.0
- 出典:スタック、StackOverFlow、GPT-3.5世代
スタック2022-11 |すべて| pl(358)| hg |論文|データセット
- 出版社:ServiceNow Research et al。
- サイズ:6 TB
- ライセンス:元のライセンスの条件
- 出典:許容式ソースコードファイル
平行コーパス
MTP 2023-9 |すべて| en&zh | Hg&ci |データセット
- 出版社:Baai
- サイズ:1.3 Tb
- ライセンス:BAAIデータ使用プロトコル
- 出典:Web上の中国語と英語の並列テキストペア
Multiun 2010-5 |すべて| Multi(7)| hg |論文| Webサイト
- 出版社:ドイツの人工知能研究センター(DFKI)GMBH
- サイズ:4353 MB
- ライセンス: -
- 出典:国連文書
Paracrawl 2020-7 |すべて| Multi(42)| hg |論文| Webサイト
- 出版社:Prompsit et al。
- サイズ:59996ファイル
- ライセンス:CC0
- 出典:Web Crawlerデータ
Uncorpus v1.0 2016-5 |すべて| Multi(6)| hg |論文| Webサイト
- 出版社:国連等。
- サイズ:799276ファイル
- ライセンス: -
- 出典:国連文書
ccaligned 2020-11 |すべて| Multi(138)| hg |論文|データセット
- 出版社:Facebook AI et al。
- サイズ:392 m URLペア
- ライセンス: -
- 出典:Common Crawl
wikimatrix 2021-4 |すべて| Multi(85)| hg |論文| github |データセット
- 出版社:Facebook AI et al。
- サイズ:134 m並列文
- ライセンス:CC-by-sa
- 出典:ウィキペディア
ソーシャルメディア
OpenWebtext 2019-4 |すべて| en | hg | Webサイト
- 出版社:ブラウン大学
- サイズ:38 GB
- ライセンス:CC0
- 出典:Reddit
Pushshift Reddit 2020-1 |すべて| en | ci |論文| Webサイト
- 出版社:Pushshift.io et al。
- サイズ:2 TB
- ライセンス: -
- 出典:Reddit
Reddit 2005-6 |すべて| en | hg | Webサイト
- 出版社:CondéNastDigital et al。
- サイズ: -
- ライセンス: -
- 出典:ソーシャルメディアの投稿
stackexchange 2008-9 |すべて| en | hg |データセット| Webサイト
- 出版社:スタック交換
- サイズ: -
- ライセンス:CC-SA-4.0
- 出典:コミュニティの質問と回答データ
webtext 2019-2 |部分的| en | hg |論文| github |データセット
- 出版社:Openai
- サイズ:40 GB
- ライセンス:MIT
- 出典:Reddit
Zhihu 2011-1 |すべて| Zh | hg | Webサイト
- 出版社:Beijing Zhizhe Tianxia Technology Co.、Ltd
- サイズ: -
- ライセンス:Zhihuユーザー契約
- 出典:ソーシャルメディアの投稿
百科事典
Baidu Baike 2008-4 |すべて| Zh | hg | Webサイト
- 出版社:Baidu
- サイズ: -
- ライセンス:Baidu Baikeユーザー契約
- 出典:百科事典コンテンツデータ
Tigerbot-wiki 2023-5 |すべて| Zh | hg |論文| github |データセット
- 出版社:Tigerbot
- サイズ:205 MB
- ライセンス:Apache-2.0
- 出典:Baidu Baike
ウィキペディア2001-1-1 |すべて|マルチ| hg |データセット| Webサイト
- 出版社:ウィキメディア財団
- サイズ: -
- ライセンス:CC-BY-SA-3.0およびGFDL
- 出典:百科事典コンテンツデータ
マルチカテゴリ
Arabictext 2022 2022-12 |すべて| ar | Hg&ci |データセット
- 出版社:Baai et al。
- サイズ:201.9 GB
- ライセンス:CC-SA-4.0
- 出典:ArabicWeb、Oscar、CC100など。
MNBVC 2023-1 |すべて| Zh | Hg&ci | github |データセット
- 出版社:Liwuコミュニティ
- サイズ:20811 GB
- ライセンス:MIT
- 出典:中国の本、ウェブページ、論文など。
redpajama-v1 2023-4 |すべて|マルチ| Hg&ci | github |データセット
- 出版社:一緒にコンピューター
- サイズ:1.2 tトークン
- ライセンス: -
- 出典:Common Crawl、Github、Booksなど。
ルーツ2023-3 |部分的| Multi(59)| Hg&ci |論文|データセット
- 出版社:Face et al。
- サイズ:1.61 TB
- ライセンス:Bloom Open-Rail-M
- 出典:オスカー、Githubなど
パイル2021-1 |すべて| en | Hg&ci |論文| github |データセット
- 出版社:Eleutherai
- サイズ:825.18 GB
- ライセンス:MIT
- 出典:書籍、arxiv、githubなど。
tigerbot_pretrain_en 2023-5 |部分的| en | ci |論文| github |データセット
- 出版社:Tigerbot
- サイズ:51 GB
- ライセンス:Apache-2.0
- 出典:英語の本、ウェブページ、en-wikiなど
tigerbot_pretrain_zh 2023-5 |部分的| Zh | hg |論文| github |データセット
- 出版社:Tigerbot
- サイズ:55 GB
- ライセンス:Apache-2.0
- 出典:中国の本、ウェブページ、Zh-wikiなど。
wanjuantext-1.0 2023-8 |すべて| Zh | hg |論文| github |データセット
- 出版社:上海AI研究所
- サイズ:1094 GB
- ライセンス:CC-By-4.0
- 出典:ウェブページ、百科事典、書籍など
DOLMA 2024-1 |すべて| en | Hg&ci |論文| github |データセット
- 出版社:AI2 et al。
- サイズ:11519 GB
- ライセンス:MR契約
- 出典:Project Gutenberg、C4、Redditなど。
Slimpajama 2023-6 |すべて| en | Hg&ci | github |データセット| Webサイト
- 出版社:Cerebras et al。
- サイズ:627 Bトークン
- ライセンス: -
- 出典:Common Crawl、C4、Githubなど。
MassiveText 2021-12 | |ではありませんマルチ| Hg&ci |紙
- 出版社:Google DeepMind
- サイズ:10.5 TB
- ライセンス: -
- 出典:MassiveWeb、C4、Booksなど
ミネルバ2022-6 | |ではありませんen | hg |紙
- 出版社:Google Research
- サイズ:38.5 bトークン
- ライセンス: -
- 出典:Arxiv、Webページなど。
MAP-CC 2024-4 |すべて| Zh | hg |論文| github |データセット| Webサイト
- 出版社:Multimodal Art Projection Research Community et al。
- サイズ:840.48 Bトークン
- ライセンス:CC-BY-NC-ND-4.0
- 出典:中国の一般的なクロール、中国百科事典、中国の本など。
Expository-Prose-V1 2024-8 |すべて| en | Hg&ci |論文| github |データセット
- 出版社:PINTS.AI LABS
- サイズ:56 Bトークン
- ライセンス:MIT
- 出典:Arxiv、Wikipedia、Gutenbergなど。
ドメイン固有のプリトレーニングコーパス
ドメイン固有のプリトレーニングコーパスは、特定のフィールドまたはトピック用にカスタマイズされたLLMデータセットです。コーパスのタイプは、通常、LLMSの漸進的なトレーニングフェーズで使用されます。コーパスは、データドメインに基づいて分類されます。
データセット情報形式:
- Dataset name Release Time | Public or Not | Language | Construction Method | Paper | Github | Dataset | Website
- Publisher:
- Size:
- License:
- Source:
- Category:
- Domain:
金融
BBT-Fincorpus 2023-2 |部分的| Zh | hg |論文| github | Webサイト
- 出版社:Fudan University et al。
- サイズ:256 GB
- ライセンス: -
- 出典:会社の発表、研究報告、財務
- カテゴリ:マルチ
- ドメイン:金融
Fincorpus 2023-9 |すべて| Zh | hg |論文| github |データセット
- 出版社:Du Xiaoman
- サイズ:60.36 GB
- ライセンス:Apache-2.0
- 出典:会社の発表、金融ニュース、金融試験の質問
- カテゴリ:マルチ
- ドメイン:金融
finglm 2023-7 |すべて| Zh | hg | github
- 出版社:知識Atlas et al。
- サイズ:69 GB
- ライセンス:Apache-2.0
- 出典:上場企業の年次報告書
- カテゴリ:言語テキスト
- ドメイン:金融
Tigerbot-hearnning 2023-5 |すべて| Zh | hg |論文| github |データセット
- 出版社:Tigerbot
- サイズ:488 MB
- ライセンス:Apache-2.0
- 出典:財務報告
- カテゴリ:言語テキスト
- ドメイン:金融
Tigerbot-Research 2023-5 |すべて| Zh | hg |論文| github |データセット
- 出版社:Tigerbot
- サイズ:696 MB
- ライセンス:Apache-2.0
- 出典:研究レポート
- カテゴリ:言語テキスト
- ドメイン:金融
医学
数学
Proof-Pile-2 2023-10 |すべて| en | Hg&ci |論文| github |データセット| Webサイト
- 出版社:プリンストン大学等。
- サイズ:55 Bトークン
- ライセンス: -
- 出典:arxiv、openwebmath、代数
- カテゴリ:マルチ
- ドメイン:数学
Mathpile 2023-12 |すべて| en | hg |論文| github |データセット
- 出版社:Shanghai Jiao Tong University et al。
- サイズ:9.5 bトークン
- ライセンス:CC-BY-NC-SA-4.0
- 出典:教科書、Wikipedia、ProofWiki、CommonCrawl、stackexchange、arxiv
- カテゴリ:マルチ
- ドメイン:数学
OpenWebMath 2023-10 |すべて| en | hg |論文| github |データセット
- 出版社:トロント大学等。
- サイズ:14.7 Bトークン
- ライセンス:ODC-1.0
- 出典:Common Crawl
- カテゴリ:ウェブページ
- ドメイン:数学
他の
指示微調整データセット
命令微調整データセットは、「命令入力」と「回答出力」を含む一連のテキストペアで構成されています。 「命令入力」は、人間がモデルに行ったリクエストを表しています。分類、要約、言い換えなど、さまざまな種類の指示があります。「回答出力」は、指示に続いて人間の期待に応じてモデルによって生成される応答です。
一般的な命令微調整データセット
一般的な命令微調整データセットには、ドメイン制限なしの1つ以上の命令カテゴリが含まれており、主に一般的なタスクでのLLMの命令にフォローする能力を強化することを目指しています。データセットは、建設方法に基づいて分類されます。
データセット情報形式:
- Dataset name Release Time | Public or Not | Language | Construction Method | Paper | Github | Dataset | Website
- Publisher:
- Size:
- License:
- Source:
- Instruction Category:
人間の生成データセット(HG)
Databricks-Dolly-15K 2023-4 |すべて| en | hg |データセット| Webサイト
- 出版社:DataBricks
- サイズ:15011インスタンス
- ライセンス:CC-SA-3.0
- 出典:さまざまな命令カテゴリに基づいて手動で生成されます
- 命令カテゴリ:マルチ
InstructionWild_V2 2023-6 |すべて| en&zh | hg | github
- 出版社:シンガポール国立大学
- サイズ:110Kインスタンス
- ライセンス: -
- 出典:Web上で収集
- 命令カテゴリ:マルチ
LCCC 2020-8 |すべて| Zh | hg |論文| github
- 出版社:Tsinghua University et al。
- サイズ:12mインスタンス
- ライセンス:MIT
- 出典:ソーシャルメディアでのユーザーインタラクションをクロールします
- 命令カテゴリ:マルチ
OASST1 2023-4 |すべて| Multi(35)| hg |論文| github |データセット
- 出版社:OpenAssistant
- サイズ:161443インスタンス
- ライセンス:Apache-2.0
- 出典:人間によって生成および注釈が付けられました
- 命令カテゴリ:マルチ
OL-CC 2023-6 |すべて| Zh | hg |データセット
- 出版社:Baai
- サイズ:11655インスタンス
- ライセンス:Apache-2.0
- 出典:人間によって生成および注釈が付けられました
- 命令カテゴリ:マルチ
Zhihu-Kol 2023-3 |すべて| Zh | hg | github |データセット
- 出版社:Wangrui6
- サイズ:1006218インスタンス
- ライセンス:MIT
- 出典:ZhihuのCrawl
- 命令カテゴリ:マルチ
AYAデータセット2024-2 |すべて| Multi(65)| hg |論文|データセット| Webサイト
- 出版社:AI Community et alのCohere。
- サイズ:204kインスタンス
- ライセンス:Apache-2.0
- 出典:AYAアノテーションプラットフォームを介して手動で収集および注釈
- 命令カテゴリ:マルチ
インストラクティ2023-5 |すべて| en&zh | hg |論文| github |データセット
- 出版社:Zhijiang University et al。
- サイズ:371700インスタンス
- ライセンス:MIT
- 出典:ウィキペディア、バイデュ・バイク
- 命令カテゴリ:抽出
モデル構築されたデータセット(MC)
alpaca_data 2023-3 |すべて| en | MC | github
- 出版社:スタンフォードアルパカ
- サイズ:52Kインスタンス
- ライセンス:Apache-2.0
- 出典:APLACA_DATAプロンプトを使用してText-Davinci-003によって生成されます
- 命令カテゴリ:マルチ
belle_generated_chat 2023-5 |すべて| Zh | MC | github |データセット
- 出版社:ベル
- サイズ:396004インスタンス
- ライセンス:GPL-3.0
- 出典:chatgptによって生成されました
- 命令カテゴリ:生成
belle_multiturn_chat 2023-5 |すべて| Zh | MC | github |データセット
- 出版社:ベル
- サイズ:831036インスタンス
- ライセンス:GPL-3.0
- 出典:chatgptによって生成されました
- 命令カテゴリ:マルチ
belle_train_0.5m_cn 2023-4 |すべて| Zh | MC | github |データセット
- 出版社:ベル
- サイズ:519255インスタンス
- ライセンス:GPL-3.0
- 出典:Text-Davinci-003によって生成されました
- 命令カテゴリ:マルチ
belle_train_1m_cn 2023-4 |すべて| Zh | MC | github |データセット
- 出版社:ベル
- サイズ:917424インスタンス
- ライセンス:GPL-3.0
- 出典:Text-Davinci-003によって生成されました
- 命令カテゴリ:マルチ
belle_train_2m_cn 2023-5 |すべて| Zh | MC | github |データセット
- 出版社:ベル
- サイズ:2mインスタンス
- ライセンス:GPL-3.0
- 出典:chatgptによって生成されました
- 命令カテゴリ:マルチ
belle_train_3.5m_cn 2023-5 |すべて| Zh | MC | github |データセット
- 出版社:ベル
- サイズ:3606402インスタンス
- ライセンス:GPL-3.0
- 出典:chatgptによって生成されました
- 命令カテゴリ:マルチ
キャメル2023-3 |すべて| Multi&pl | MC |論文| github |データセット| Webサイト
- 出版社:カウスト
- サイズ:1659328インスタンス
- ライセンス:CC-BY-NC-4.0
- 出典:2つのGPT-3.5ターボエージェントによって生成されたダイアログ
- 命令カテゴリ:マルチ
chatgpt_corpus 2023-6 |すべて| Zh | MC | github
- 出版社:Plexpt
- サイズ:3270Kインスタンス
- ライセンス:GPL-3.0
- 出典:GPT-3.5-Turboによって生成されました
- 命令カテゴリ:マルチ
InstructionWild_V1 2023-3 |すべて| en&zh | MC | github
- 出版社:シンガポール国立大学
- サイズ:104kインスタンス
- ライセンス: -
- 出典:Openai APIによって生成されました
- 命令カテゴリ:マルチ
lmsys-chat-1M 2023-9 |すべて|マルチ| MC |論文|データセット
- 出版社:UC Berkeley et al。
- サイズ:1Mインスタンス
- ライセンス:LMSYS-chat-1Mライセンス
- 出典:複数のLLMによって生成されます
- 命令カテゴリ:マルチ
MOSS_002_SFT_DATA 2023-4 |すべて| en&zh | MC | github |データセット
- 出版社:Fudan University
- サイズ:1161137インスタンス
- ライセンス:CC-BY-NC-4.0
- 出典:Text-Davinci-003によって生成されました
- 命令カテゴリ:マルチ
MOSS_003_SFT_DATA 2023-4 |すべて| en&zh | MC | github |データセット
- 出版社:Fudan University
- サイズ:1074551インスタンス
- ライセンス:CC-BY-NC-4.0
- 出典:MOSS-002からの会話データとGPT-3.5-Turboによって生成されました
- 命令カテゴリ:マルチ
moss_003_sft_plugin_data 2023-4 |部分的| en&zh | MC | github |データセット
- 出版社:Fudan University
- サイズ:300Kインスタンス
- ライセンス:CC-BY-NC-4.0
- 出典:プラグインとLLMによって生成されます
- 命令カテゴリ:マルチ
OpenChat 2023-7 |すべて| en | MC |論文| github |データセット
- 出版社:Tsinghua University et al。
- サイズ:70Kインスタンス
- ライセンス:MIT
- 出典:sharegpt
- 命令カテゴリ:マルチ
redgpt-dataset-v1-cn 2023-4 |部分的| Zh | MC | github
- 出版社:Da-Southampton
- サイズ:50kインスタンス
- ライセンス:Apache-2.0
- 出典:LLMSによって生成されました
- 命令カテゴリ:マルチ
自己内容2022-12 |すべて| en | MC |論文| github
- 出版社:ワシントン大学等。
- サイズ:52445インスタンス
- ライセンス:Apache-2.0
- 出典:GPT-3によって生成されました
- 命令カテゴリ:マルチ
ShareChat 2023-4 |すべて|マルチ| MC | Webサイト
- 出版社:Sharechat
- サイズ:90Kインスタンス
- ライセンス:CC0
- 出典:sharegpt
- 命令カテゴリ:マルチ
sharegpt-chinese-english-90k 2023-7 |すべて| en&zh | MC | github |データセット
- 出版社:Shareai
- サイズ:90Kインスタンス
- ライセンス:Apache-2.0
- 出典:sharegpt
- 命令カテゴリ:マルチ
Sharegpt90k 2023-4 |すべて| en | MC |データセット
- 出版社:Ryokoai
- サイズ:90Kインスタンス
- ライセンス:CC0
- 出典:sharegpt
- 命令カテゴリ:マルチ
Ultrachat 2023-5 |すべて| en | MC |論文| github
- 出版社:ツィンフア大学
- サイズ:1468352インスタンス
- ライセンス:CC-BY-NC-4.0
- 出典:2つのChatGPTエージェントによって生成されたダイアログ
- 命令カテゴリ:マルチ
不自然な指示2022-12 |すべて| en | MC |論文| github
- 出版社:Tel Aviv University et al。
- サイズ:240670インスタンス
- ライセンス:MIT
- 出典:LLMSによって生成されました
- 命令カテゴリ:マルチ
webglm-qa 2023-6 |すべて| en | MC |論文| github |データセット
- 出版社:Tsinghua University et al。
- サイズ:44979インスタンス
- ライセンス:Apache-2.0
- 出典:LLM内のコンテキストブートストラップを介してWebGlm-QAを構築します
- 命令カテゴリ:QAを開きます
wizard_evol_instruct_196k 2023-6 |すべて| en | MC |論文| github |データセット
- 出版社:Microsoft et al。
- サイズ:196kインスタンス
- ライセンス: -
- 出典:Evol-Instructメソッドを介して命令を進化させます
- 命令カテゴリ:マルチ
wizard_evol_instruct_70k 2023-5 |すべて| en | MC |論文| github |データセット
- 出版社:Microsoft et al。
- サイズ:70Kインスタンス
- ライセンス: -
- 出典:Evol-Instructメソッドを介して命令を進化させます
- 命令カテゴリ:マルチ
WildChat 2024-5 |部分的|マルチ| MC |論文|データセット
- 出版社:Cornell University et al。
- サイズ:1039785インスタンス
- ライセンス:AI2インパクトライセンス
- 出典:ユーザーとChatGpt、GPT-4の間の会話
- 命令カテゴリ:マルチ
既存のデータセット(CI)の収集と改善
CrossFit 2021-4 |すべて| en | ci |論文| github
- 出版社:南カリフォルニア大学
- サイズ:269データセット
- ライセンス: -
- 出典:さまざまなNLPデータセットの収集と改善
- 命令カテゴリ:マルチ
Dialogstudio 2023-7 |すべて| en | ci |論文| github |データセット
- 出版社:Salesforce Ai et al。
- サイズ:87データセット
- ライセンス:Apache-2.0
- 出典:さまざまなNLPデータセットの収集と改善
- 命令カテゴリ:マルチ
Dynosaur2023-5 |すべて| en | ci |論文| github |データセット| Webサイト
- 出版社:UCLA et al。
- サイズ:801900インスタンス
- ライセンス:Apache-2.0
- 出典:さまざまなNLPデータセットの収集と改善
- 命令カテゴリ:マルチ
Flan-Mini 2023-7 |すべて| en | ci |論文| github |データセット
- 出版社:シンガポール工科大学デザイン
- サイズ:1.34mインスタンス
- ライセンス:CC
- 出典:さまざまな命令の微調整データセットの収集と改善
- 命令カテゴリ:マルチ
Flan 2021 2021-9 |すべて|マルチ| ci |論文| github
- 出版社:Google Research
- サイズ:62データセット
- ライセンス:Apache-2.0
- 出典:さまざまなNLPデータセットの収集と改善
- 命令カテゴリ:マルチ
Flan 2022 2023-1 |部分的|マルチ| ci |論文| github |データセット
- 出版社:Google Research
- サイズ:1836データセット
- ライセンス:Apache-2.0
- 出典:さまざまな命令の微調整データセットの収集と改善
- 命令カテゴリ:マルチ
Instructdial 2022-5 |すべて| en | ci |論文| github
- 出版社:カーネギーメロン大学
- サイズ:59データセット
- ライセンス:Apache-2.0
- 出典:さまざまなNLPデータセットの収集と改善
- 命令カテゴリ:マルチ
自然指示2021-4 |すべて| en | ci |論文| github |データセット
- 出版社:AI等のアレン研究所。
- サイズ:61データセット
- ライセンス:Apache-2.0
- 出典:さまざまなNLPデータセットの収集と改善
- 命令カテゴリ:マルチ
OIG2023-3 |すべて| en | ci |データセット
- 出版社:ライオン
- サイズ:3878622インスタンス
- ライセンス:Apache-2.0
- 出典:さまざまなデータセットの収集と改善
- 命令カテゴリ:マルチ
オープンプラタイプ2023-8 |すべて| en | ci |論文| github |データセット| Webサイト
- 出版社:ボストン大学
- サイズ:24926インスタンス
- ライセンス: -
- 出典:さまざまなデータセットの収集と改善
- 命令カテゴリ:マルチ
OPT-IMLベンチ2022-12 | |ではありませんマルチ| ci |論文| github
- Publisher: Meta AI
- Size: 2000 datasets
- License: MIT
- Source: Collection and improvement of various NLP datasets
- Instruction Category: Multi
PromptSource 2022-2 | All | EN | CI | Paper | github
- Publisher: Brown University et al.
- Size: 176 datasets
- License: Apache-2.0
- Source: Collection and improvement of various NLP datasets
- Instruction Category: Multi
SUPER-NATURAL INSTRUCTIONS 2022-4 | All | Multi | CI | Paper | github
- Publisher: Univ. of Washington et al.
- Size: 1616 datasets
- License: Apache-2.0
- Source: Collection and improvement of various NLP datasets
- Instruction Category: Multi
T0 2021-10 | All | EN | CI | Paper | Dataset1 | Dataset2
- Publisher: Hugging Face et al.
- Size: 62 datasets
- License: Apache-2.0
- Source: Collection and improvement of various NLP datasets
- Instruction Category: Multi
UnifiedSKG 2022-3 | All | EN | CI | Paper | github
- Publisher: The University of Hong Kong et al.
- Size: 21 datasets
- License: Apache-2.0
- Source: Collection and improvement of various NLP datasets
- Instruction Category: Multi
xP3 2022-11 | All | Multi (46) | CI | Paper | github
- Publisher: Hugging Face et al.
- Size: 82 datasets
- License: Apache-2.0
- Source: Collection and improvement of various NLP datasets
- Instruction Category: Multi
IEPile 2024-2 | All | EN & ZH | CI | Paper | Github |データセット
- Publisher: Zhejiang University et al.
- Size: 33 datasets
- License: CC-BY-NC-SA-4.0
- Source: Collection and improvement of various IE datasets
- Instruction Category: Extraction
KOLLM-Conversations 2024-3 | All | KO | CI |データセット
- Publisher: davidkim205
- Size: 1122566 instances
- License: Apache-2.0
- Source: Collection and improvement of Korean datasets
- Instruction Category: Multi
HG & CI
Firefly 2023-4 | All | ZH | HG & CI | Github |データセット
- Publisher: YeungNLP
- Size: 1649399 instances
- License: -
- Source: Collect Chinese NLP datasets and manually generate data related to Chinese culture
- Instruction Category: Multi
LIMA-sft 2023-5 | All | EN | HG & CI | Paper |データセット
- Publisher: Meta AI et al.
- Size: 1330 instances
- License: CC-BY-NC-SA
- Source: Manually select from various types of data
- Instruction Category: Multi
COIG-CQIA 2024-3 | All | ZH | HG & CI | Paper |データセット
- Publisher: Shenzhen Institute of Advanced Technology et al.
- Size: 48375 instances
- License: -
- Source: Q&A communities, Wikipedia, examinations, existing NLP datasets
- Instruction Category: Multi
HG & MC
- InstructGPT-sft 2022-3 | Not | EN | HG & MC |紙
- Publisher: OpenAI
- Size: 14378 instances
- License: -
- Source: Platform Q&A data and manual labeling
- Instruction Category: Multi
CI & MC
Alpaca_GPT4_data 2023-4 | All | EN | CI & MC | Paper | github
- Publisher: Microsoft Research
- Size: 52K instances
- License: Apache-2.0
- Source: Generated by GPT-4 with Aplaca_data prompts
- Instruction Category: Multi
Alpaca_GPT4_data_zh 2023-4 | All | ZH | CI & MC | Github |データセット
- Publisher: Microsoft Research
- Size: 52K instances
- License: Apache-2.0
- Source: Generated by GPT-4 with Alpaca_data prompts translated into Chinese by ChatGPT
- Instruction Category: Multi
Bactrain-X 2023-5 | All | Multi (52) | CI & MC | Paper | Github |データセット
- Publisher: MBZUAI
- Size: 3484884 instances
- License: CC-BY-NC-4.0
- Source: Generated by GPT-3.5-Turbo with Aplaca_data and databricks-dolly-15K prompts translated into 51 languages by Google Translate API
- Instruction Category: Multi
Baize 2023-3 | Partial | EN | CI & MC | Paper | Github |データセット
- Publisher: University of California et al.
- Size: 210311 instances
- License: GPL-3.0
- Source: Sample seeds from specific datasets to create multi-turn dialogues using ChatGPT
- Instruction Category: Multi
GPT4All 2023-3 | All | EN | CI & MC | Paper | Github |データセット
- Publisher: nomic-ai
- Size: 739259 instances
- License: MIT
- Source: Generated by GPT-3.5-Turbo with other datasets' prompts
- Instruction Category: Multi
GuanacoDataset 2023-3 | All | Multi | CI & MC | Dataset | Webサイト
- Publisher: JosephusCheung
- Size: 534530 instances
- License: GPL-3.0
- Source: Expand upon the initial 52K dataset from the Alpaca model
- Instruction Category: Multi
LaMini-LM 2023-4 | All | EN | CI & MC | Paper | Github |データセット
- Publisher: Monash University et al.
- Size: 2585615 instances
- License: CC-BY-NC-4.0
- Source: Generated by ChatGPT with synthetic and existing prompts
- Instruction Category: Multi
LogiCoT 2023-5 | All | EN & ZH | CI & MC | Paper | Github |データセット
- Publisher: Westlake University et al.
- Size: 604840 instances
- License: CC-BY-NC-ND-4.0
- Source: Expand the datasets using GPT-4
- Instruction Category: Reasoning
LongForm 2023-4 | All | EN | CI & MC | Paper | Github |データセット
- Publisher: LMU Munich et al.
- Size: 27739 instances
- License: MIT
- Source: Select documents from existing corpora and generating prompts for the documents using LLMs
- Instruction Category: Multi
Luotuo-QA-B 2023-5 | All | EN & ZH | CI & MC | Github |データセット
- Publisher: Luotuo
- Size: 157320 instances
- License: Apache-2.0 & CC0
- Source: Use LLMs to generate Q&A pairs on CSL, arXiv, and CNN-DM datasets
- Instruction Category: Multi
OpenOrca 2023-6 | All | Multi | CI & MC | Paper |データセット
- Publisher: Microsoft Researc
- Size: 4233923 instances
- License: MIT
- Source: Expand upon the Flan 2022 dataset using GPT-3.5-Turbo and GPT-4
- Instruction Category: Multi
Wizard_evol_instruct_zh 2023-5 | All | ZH | CI & MC | Github |データセット
- Publisher: Central China Normal University et al.
- Size: 70K instances
- License: CC-BY-4.0
- Source: Generated by GPT with Wizard_evol_instruct prompts translated into Chinese
- Instruction Category: Multi
Lithuanian-QA-v1 2024-8 | All | LT | CI & MC | Paper |データセット
- Publisher: Neurotechnology
- Size: 13848 instances
- License: CC-BY-4.0
- Source: Use ChatGPT to generate Q&A pairs on Wikipedia corpus
- Instruction Category: Multi
LongWriter-6K 2024-8 | All | EN & ZH | CI & MC | Paper | Github |データセット
- Publisher: Tsinghua University et al.
- Size: 6000 instances
- License: Apache-2.0
- Source: Generated by GPT-4o with open-source datasets' prompts
- Instruction Category: Multi
HG & CI & MC
COIG 2023-4 | All | ZH | HG & CI & MC | Paper | Github |データセット
- Publisher: BAAI
- Size: 191191 instances
- License: Apache-2.0
- Source: Translated instructions, Leetcode, Chinese exams, etc.
- Instruction Category: Multi
HC3 2023-1 | All | EN & ZH | HG & CI & MC | Paper | Github | Dataset1 | Dataset2
- Publisher: SimpleAI
- Size: 37175 instances
- License: CC-BY-SA-4.0
- Source: Human-Q&A pairs and ChatGPT-Q&A pairs from Q&A platforms, encyclopedias, etc.
- Instruction Category: Multi
Phoenix-sft-data-v1 2023-5 | All | Multi | HG & CI & MC | Paper | Github |データセット
- Publisher: The Chinese University of Hong Kong et al.
- Size: 464510 instances
- License: CC-BY-4.0
- Source: Collected multi-lingual instructions, post-translated multi-lingual instructions, self-generated user-centered multi-lingual instructions
- Instruction Category: Multi
TigerBot_sft_en 2023-5 | Partial | EN | HG & CI & MC | Paper | Github |データセット
- Publisher: TigerBot
- Size: 677117 instances
- License: Apache-2.0
- Source: Self-instruct, human-labeling, open-source data cleaning
- Instruction Category: Multi
TigerBot_sft_zh 2023-5 | Partial | ZH | HG & CI & MC | Paper | Github |データセット
- Publisher: TigerBot
- Size: 530705 instances
- License: Apache-2.0
- Source: Self-instruct, human-labeling, open-source data cleaning
- Instruction Category: Multi
Aya Collection 2024-2 | All | Multi (114) | HG & CI & MC | Paper | Dataset | Webサイト
- Publisher: Cohere For AI Community et al.
- Size: 513M instances
- License: Apache-2.0
- Source: Templated data, Translated data and Aya Dataset
- Instruction Category: Multi
REInstruct 2024-8 | Not | EN | HG & CI & MC | Paper | github
- Publisher: Chinese Information Processing Laboratory et al.
- Size: 35K instances
- License: -
- Source: Automatically constructing instruction data from the C4 corpus using a small amount of manually annotated seed instruction data
- Instruction Category: Multi
Domain-specific Instruction Fine-tuning Datasets
The domain-specific instruction fine-tuning datasets are constructed for a particular domain by formulating instructions that encapsulate knowledge and task types closely related to that domain.
Dataset information format:
- Dataset name Release Time | Public or Not | Language | Construction Method | Paper | Github | Dataset | Website
- Publisher:
- Size:
- License:
- Source:
- Instruction Category:
- Domain:
医学
ChatDoctor 2023-3 | All | EN | HG & MC | Paper | Github |データセット
- Publisher: University of Texas Southwestern Medical Center et al.
- Size: 115K instances
- License: Apache-2.0
- Source: Real conversations between doctors and patients & Generated by ChatGPT
- Instruction Category: Multi
- Domain: Medical
ChatMed_Consult_Dataset 2023-5 | All | ZH | MC | Github |データセット
- Publisher: michael-wzhu
- Size: 549326 instances
- License: CC-BY-NC-4.0
- Source: Generated by GPT-3.5-Turbo
- Instruction Category: Multi
- Domain: Medical
CMtMedQA 2023-8 | All | ZH | HG | Paper | Github |データセット
- Publisher: Zhengzhou University
- Size: 68023 instances
- License: MIT
- Source: Real conversations between doctors and patients
- Instruction Category: Multi
- Domain: Medical
DISC-Med-SFT 2023-8 | All | ZH | HG & CI | Paper | Github | Dataset | Webサイト
- Publisher: Fudan University et al.
- Size: 464898 instances
- License: Apache-2.0
- Source: Open source datasets & Manually selected data
- Instruction Category: Multi
- Domain: Medical
HuatuoGPT-sft-data-v1 2023-5 | All | ZH | HG & MC | Paper | Github |データセット
- Publisher: The Chinese University of Hong Kong et al.
- Size: 226042 instances
- License: Apache-2.0
- Source: Real conversations between doctors and patients & Generated by ChatGPT
- Instruction Category: Multi
- Domain: Medical
Huatuo-26M 2023-5 | Partial | ZH | CI | Paper | github
- Publisher: The Chinese University of Hong Kong et al.
- Size: 26504088 instances
- License: Apache-2.0
- Source: Collection and improvement of various datasets
- Instruction Category: Multi
- Domain: Medical
MedDialog 2020-4 | All | EN & ZH | HG | Paper | github
- Publisher: UC San Diego
- Size: 3.66M instances
- License: -
- Source: Real conversations between doctors and patients
- Instruction Category: Multi
- Domain: Medical
Medical Meadow 2023-4 | All | EN | HG & CI | Paper | Github |データセット
- Publisher: University Hospital Aachen et al.
- Size: 160076 instances
- License: GPL-3.0
- Source: Crawl data from the Internet & Collection and improvement of various NLP datasets
- Instruction Category: Multi
- Domain: Medical
Medical-sft 2023-5 | All | EN & ZH | CI | Github |データセット
- Publisher: Ming Xu
- Size: 2.07M instances
- License: Apache-2.0
- Source: Collection and improvement of various NLP datasets
- Instruction Category: Multi
- Domain: Medical
QiZhenGPT-sft-20k 2023-5 | Partial | ZH | CI | Github |データセット
- Publisher: Zhejiang University
- Size: 20K instances
- License: GPL-3.0
- Source: Collection and improvement of various datasets
- Instruction Category: Multi
- Domain: Medical
ShenNong_TCM_Dataset 2023-6 | All | ZH | MC | Github |データセット
- Publisher: michael-wzhu
- Size: 112565 instances
- License: Apache-2.0
- Source: Generated by ChatGPT
- Instruction Category: Multi
- Domain: Medical
コード
Code_Alpaca_20K 2023-3 | All | EN & PL | MC | Github |データセット
- Publisher: Sahil Chaudhary
- Size: 20K instances
- License: Apache-2.0
- Source: Generated by Text-Davinci-003
- Instruction Category: Code
- Domain: Code
CodeContest 2022-3 | All | EN & PL | CI | Paper | github
- Publisher: DeepMind
- Size: 13610 instances
- License: Apache-2.0
- Source: Collection and improvement of various datasets
- Instruction Category: Code
- Domain: Code
CommitPackFT 2023-8 | All | EN & PL (277) | HG | Paper | Github |データセット
- Publisher: Bigcode
- Size: 702062 instances
- License: MIT
- Source: GitHub Action dump
- Instruction Category: Code
- Domain: Code
ToolAlpaca 2023-6 | All | EN & PL | HG & MC | Paper | github
- Publisher: Chinese Information Processing Laboratory et al.
- Size: 3928 instances
- License: Apache-2.0
- Source: Manually filter APIs & Generated by ChatGPT
- Instruction Category: Code
- Domain: Code
ToolBench 2023-7 | All | EN & PL | HG & MC | Paper | github
- Publisher: Tsinghua University et al.
- Size: 126486 instances
- License: Apache-2.0
- Source: Manually filter APIs & Generated by ChatGPT
- Instruction Category: Code
- Domain: Code
法律上の
DISC-Law-SFT 2023-9 | Partial | ZH | HG & CI & MC | Paper | Github | Webサイト
- Publisher: Fudan University et al.
- Size: 403K instances
- License: Apache-2.0
- Source: Open source datasets & Legal-related Text Content & Generated by GPT-3.5-Turbo
- Instruction Category: Multi
- Domain: Law
HanFei 1.0 2023-5 | All | ZH | - | Github |データセット
- Publisher: Chinese Academy of Sciences et al.
- Size: 255K instances
- License: Apache-2.0
- Source: Filter legal-related data according to rules
- Instruction Category: Multi
- Domain: Law
LawGPT_zh 2023-5 | Partial | ZH | CI & MC | Github |データセット
- Publisher: Shanghai Jiao Tong University
- Size: 200K instances
- License: -
- Source: Real conversations & Generated by ChatGPT
- Instruction Category: Multi
- Domain: Law
Lawyer LLaMA_sft 2023-5 | Partial | ZH | CI & MC | Paper | Github |データセット
- Publisher: Peking Universit
- Size: 21476 instances
- License: Apache-2.0
- Source: Generated by ChatGPT with other datasets' prompts
- Instruction Category: Multi
- Domain: Law
数学
BELLE_School_Math 2023-5 | All | ZH | MC | Github |データセット
- Publisher: BELLE
- Size: 248481 instances
- License: GPL-3.0
- Source: Generated by ChatGPT
- Instruction Category: Math
- Domain: Math
Goat 2023-5 | All | EN | HG | Paper | Github |データセット
- Publisher: National University of Singapore
- Size: 1746300 instances
- License: Apache-2.0
- Source: Artificially synthesized data
- Instruction Category: Math
- Domain: Math
MWP 2021-9 | All | EN & ZH | CI | Paper | Github |データセット
- Publisher: Xihua University et al.
- Size: 251598 instances
- License: MIT
- Source: Collection and improvement of various datasets
- Instruction Category: Math
- Domain: Math
OpenMathInstruct-1 2024-2 | All | EN | CI & MC | Paper | Github |データセット
- Publisher: NVIDIA
- Size: 1.8M instances
- License: NVIDIA License
- Source: GSM8K and MATH datasets (original questions); Generated using Mixtral-8×7B model
- Instruction Category: Math
- Domain: Math
教育
Child_chat_data 2023-8 | All | ZH | HG & MC | github
- Publisher: Harbin Institute of Technology et al.
- Size: 5000 instances
- License: -
- Source: Real conversations & Generated by GPT-3.5-Turbo
- Instruction Category: Multi
- Domain: Education
Educhat-sft-002-data-osm 2023-7 | All | EN & ZH | CI | Paper | Github |データセット
- Publisher: East China Normal University et al.
- Size: 4279419 instances
- License: CC-BY-NC-4.0
- Source: Collection and improvement of various datasets
- Instruction Category: Multi
- Domain: Education
TaoLi_data 2023-X | All | ZH | HG & CI | Github |データセット
- Publisher: Beijing Language and Culture University et al.
- Size: 88080 instances
- License: Apache-2.0
- Source: Collection and improvement of various datasets & Manually extract dictionary data
- Instruction Category: Multi
- Domain: Education
他の
DISC-Fin-SFT 2023-10 | Partial | ZH | HG & CI & MC | Paper | Github | Webサイト
- Publisher: Fudan University et al.
- Size: 246K instances
- License: Apache-2.0
- Source: Open source datasets & Manually collect financial data & ChatGPT assistance
- Instruction Category: Multi
- Domain: Financial
AlphaFin 2024-3 | All | EN & ZH | HG & CI & MC | Paper | Github |データセット
- Publisher: South China University of Technology et al.
- Size: 167362 instances
- License: Apache-2.0
- Source: Traditional research datasets, real-time financial data, handwritten CoT data
- Instruction Category: Multi
- Domain: Financial
GeoSignal 2023-6 | Partial | EN | HG & CI & MC | Paper | Github |データセット
- Publisher: Shanghai Jiao Tong University et al.
- Size: 22627272 instances
- License: Apache-2.0
- Source: Open source datasets & Geoscience-related Text Content & Generated by GPT-4
- Instruction Category: Multi
- Domain: Geoscience
MeChat 2023-4 | All | ZH | CI & MC | Paper | Github |データセット
- Publisher: Zhejiang University et al.
- Size: 56K instances
- License: CC0-1.0
- Source: Based on PsyQA dataset with the proposed SMILE method
- Instruction Category: Multi
- Domain: Mental Health
Mol-Instructions 2023-6 | All | EN | HG & CI & MC | Paper | Github |データセット
- Publisher: Zhejiang University et al.
- Size: 2043586 instances
- License: CC-BY-4.0
- Source: Molecule-oriented, Protein-oriented, Biomolecular text instructions
- Instruction Category: Multi
- Domain: Biology
Owl-Instruction 2023-9 | All | EN & ZH | HG & MC | Paper | github
- Publisher: Beihang University et al.
- Size: 17858 instances
- License: -
- Source: Generated by GPT-4 & Manual verification
- Instruction Category: Multi
- Domain: IT
PROSOCIALDIALOG 2022-5 | All | EN | HG & MC | Paper |データセット
- Publisher: Allenai
- Size: 165681 instances
- License: CC-BY-4.0
- Source: Generated by humans with GPT-3 created prompts
- Instruction Category: Social Norms
- Domain: Social Norms
TransGPT-sft 2023-7 | All | ZH | HG | Github |データセット
- Publisher: Beijing Jiaotong University
- Size: 58057 instances
- License: Apache-2.0
- Source: Manually collect traffic-related data
- Instruction Category: Multi
- Domain: Transportation
Preference Datasets
Preference datasets are collections of instructions that provide preference evaluations for multiple responses to the same instruction input.
Preference Evaluation Methods
The preference evaluation methods for preference datasets can be categorized into voting, sorting, scoring, and other methods. Datasets are classified based on preference evaluation methods.
Dataset information format:
- Dataset name Release Time | Public or Not | Language | Construction Method | Paper | Github | Dataset | Website
- Publisher:
- Size:
- License:
- Domain:
- Instruction Category:
- Preference Evaluation Method:
- Source:
投票する
Chatbot_arena_conversations 2023-6 | All | Multi | HG & MC | Paper |データセット
- Publisher: UC Berkeley et al.
- Size: 33000 instances
- License: CC-BY-4.0 & CC-BY-NC-4.0
- Domain: General
- Instruction Category: Multi
- Preference Evaluation Method: VO-H
- Source: Generated by twenty LLMs & Manual judgment
hh-rlhf 2022-4 | All | EN | HG & MC | Paper1 | Paper2 | Github |データセット
- Publisher: Anthropic
- Size: 169352 instances
- License: MIT
- Domain: General
- Instruction Category: Multi
- Preference Evaluation Method: VO-H
- Source: Generated by LLMs & Manual judgment
MT-Bench_human_judgments 2023-6 | All | EN | HG & MC | Paper | Github | Dataset | Webサイト
- Publisher: UC Berkeley et al.
- Size: 3.3K instances
- License: CC-BY-4.0
- Domain: General
- Instruction Category: Multi
- Preference Evaluation Method: VO-H
- Source: Generated by LLMs & Manual judgment
PKU-SafeRLHF 2023-7 | Partial | EN | HG & CI & MC | Paper | Github |データセット
- Publisher: Peking University
- Size: 361903 instances
- License: CC-BY-NC-4.0
- Domain: Social Norms
- Instruction Category: Social Norms
- Preference Evaluation Method: VO-H
- Source: Generated by LLMs & Manual judgment
SHP 2021-10 | All | EN | HG | Paper | Github |データセット
- Publisher: Stanford
- Size: 385563 instances
- License: -
- Domain: General
- Instruction Category: Multi
- Preference Evaluation Method: VO-H
- Source: Reddit data & Manual judgment
Zhihu_rlhf_3k 2023-4 | All | ZH | HG |データセット
- Publisher: Liyucheng
- Size: 3460 instances
- License: CC-BY-2.0
- Domain: General
- Instruction Category: Multi
- Preference Evaluation Method: VO-H
- Source: Zhihu data & Manual judgment
Summarize_from_Feedback 2020-9 | All | EN | HG & CI | Paper |データセット
- Publisher: OpenAI
- Size: 193841 instances
- License: -
- Domain: News
- Instruction Category: Multi
- Preference Evaluation Method: VO-H & SC-H
- Source: Open source datasets & Manual judgment and scoring
CValues 2023-7 | All | ZH | MC | Paper | Github |データセット
- Publisher: Alibaba Group
- Size: 145K instances
- License: Apache-2.0
- Domain: Social Norms
- Instruction Category: Social Norms
- Preference Evaluation Method: VO-M
- Source: Generated by LLMs & Evaluation by the reward model
huozi_rlhf_data 2024-2 | All | ZH | HG & MC | Github |データセット
- Publisher: Huozi-Team
- Size: 16918 instances
- License: Apache-2.0
- Domain: General
- Instruction Category: Multi
- Preference Evaluation Method: VO-H
- Source: Generated by Huozi model & Manual judgment
選別
- OASST1_pairwise_rlhf_reward 2023-5 | All | Multi | CI |データセット
- Publisher: Tasksource
- Size: 18918 instances
- License: Apache-2.0
- Domain: General
- Instruction Category: Multi
- Preference Evaluation Method: SO-H
- Source: OASST1 datasets & Manual sorting
スコア
Stack-Exchange-Preferences 2021-12 | All | EN | HG | Paper |データセット
- Publisher: Anthropic
- Size: 10807695 instances
- License: CC-BY-SA-4.0
- Domain: General
- Instruction Category: Multi
- Preference Evaluation Method: SC-H
- Source: Stackexchange data & Manual scoring
WebGPT 2021-12 | All | EN | HG & CI | Paper |データセット
- Publisher: OpenAI
- Size: 19578 instances
- License: -
- Domain: General
- Instruction Category: Multi
- Preference Evaluation Method: SC-H
- Source: Open source datasets & Manual scoring
Alpaca_comparison_data 2023-3 | All | EN | MC | github
- Publisher: Stanford Alpaca
- Size: 51K instances
- License: Apache-2.0
- Domain: General
- Instruction Category: Multi
- Preference Evaluation Method: SC-M
- Source: Generated by three LLMs & GPT-4 scoring
Stable_Alignment 2023-5 | All | EN | MC | Paper | github
- Publisher: Dartmouth College et al.
- Size: 169K instances
- License: Apache-2.0
- Domain: General
- Instruction Category: Multi
- Preference Evaluation Method: SC-M
- Source: Generated by LLMs & Model scoring
UltraFeedback 2023-10 | All | EN | CI & MC | Paper | Github |データセット
- Publisher: Tsinghua University et al.
- Size: 63967 instances
- License: MIT
- Domain: General
- Instruction Category: Multi
- Preference Evaluation Method: SC-M
- Source: Generated by seventeen LLMs & Model scoring
OpenHermesPreferences 2024-2 | All | EN | CI & MC |データセット
- Publisher: Argilla et al.
- Size: 989490 instances
- License: -
- Domain: General
- Instruction Category: Multi
- Preference Evaluation Method: SO-M
- Source: OpenHermes-2.5 dataset & Model sorting
HelpSteer 2023-11 | All | EN | HG & CI & MC | Paper |データセット
- Publisher: NVIDIA
- Size: 37120 instances
- License: CC-BY-4.0
- Domain: General
- Instruction Category: Multi
- Preference Evaluation Method: SC-H
- Source: Generated by LLMs & Manual judgment
HelpSteer2 2024-6 | All | EN | HG & CI & MC | Paper | Github |データセット
- Publisher: NVIDIA
- Size: 21362 instances
- License: CC-BY-4.0
- Domain: General
- Instruction Category: Multi
- Preference Evaluation Method: SC-H
- Source: Generated by LLMs & Manual judgment
他の
Evaluation Datasets
Evaluation datasets are a carefully curated and annotated set of data samples used to assess the performance of LLMs across various tasks. Datasets are classified based on evaluation domains.
Dataset information format:
- Dataset name Release Time | Public or Not | Language | Construction Method | Paper | Github | Dataset | Website
- Publisher:
- Size:
- License:
- Question Type:
- Evaluation Method:
- Focus:
- Numbers of Evaluation Categories/Subcategories:
- Evaluation Category:
一般的な
AlpacaEval 2023-5 | All | EN | CI & MC | Paper | Github | Dataset | Webサイト
- Publisher: Stanford et al.
- Size: 805 instances
- License: Apache-2.0
- Question Type: SQ
- Evaluation Method: ME
- Focus: The performance on open-ended question answering
- Numbers of Evaluation Categories/Subcategories: 1/-
- Evaluation Category: Open-ended question answering
BayLing-80 2023-6 | All | EN & ZH | HG & CI | Paper | Github |データセット
- Publisher: Chinese Academy of Sciences
- Size: 320 instances
- License: GPL-3.0
- Question Type: SQ
- Evaluation Method: ME
- Focus: Chinese-English language proficiency and multimodal interaction skills
- Numbers of Evaluation Categories/Subcategories: 9/-
- Evaluation Category: Writing, Roleplay, Common-sense, Fermi, Counterfactual, Coding, Math, Generic, Knowledge
BELLE_eval 2023-4 | All | ZH | HG & MC | Paper | github
- Publisher: BELLE
- Size: 1000 instances
- License: Apache-2.0
- Question Type: SQ
- Evaluation Method: ME
- Focus: The performance of Chinese language models in following instructions
- Numbers of Evaluation Categories/Subcategories: 9/-
- Evaluation Category: Extract, Closed qa, Rewrite, Summarization, Generation, Classification, Brainstorming, Open qa, Others
CELLO 2023-9 | All | EN | HG | Paper | github
- Publisher: Fudan University et al.
- Size: 523 instances
- License: -
- Question Type: SQ
- Evaluation Method: CE
- Focus: The ability of LLMs to understand complex instructions
- Numbers of Evaluation Categories/Subcategories: 2/10
- Evaluation Category: Complex task description, Complex input
MT-Bench 2023-6 | All | EN | HG | Paper | Github | Webサイト
- Publisher: UC Berkeley et al.
- Size: 80 instances
- License: Apache-2.0
- Question Type: SQ
- Evaluation Method: ME
- Focus: The performance on open-ended question answering
- Numbers of Evaluation Categories/Subcategories: 8/-
- Evaluation Category: Writing, Roleplay, Reasoning, Math, Coding, Extraction, STEM, Humanities
SuperCLUE 2023-7 | Not | ZH | HG & MC | Paper | Github | Website1 | Website2
- Publisher: CLUE et al.
- Size: 3754 instances
- License: -
- Question Type: Multi
- Evaluation Method: HE & CE
- Focus: The performance in a Chinese context
- Numbers of Evaluation Categories/Subcategories: 2/-
- Evaluation Category: Open multi-turn open questions, OPT objective questions
Vicuna Evaluation 2023-3 | All | EN | HG | Github | Dataset | Webサイト
- Publisher: LMSYS ORG
- Size: 80 instances
- License: Apache-2.0
- Question Type: SQ
- Evaluation Method: ME
- Focus: The performance on open-ended question answering
- Numbers of Evaluation Categories/Subcategories: 9/-
- Evaluation Category: Generic, Knowledge, Roleplay, Common-sense, Fermi, Counterfactual, Coding, Math, Writing
CHC-Bench 2024-4 | All | ZH | HG & CI | Paper | Github | Dataset | Webサイト
- Publisher: Multimodal Art Projection Research Community et al.
- Size: 214 instances
- License: Apache-2.0
- Question Type: Multi
- Evaluation Method: ME
- Focus: Hard-case Chinese instructions understanding and following
- Numbers of Evaluation Categories/Subcategories: 8/-
- Evaluation Category: Writing, Humanity, Science, Role-playing, Reading Comprehension, Math, Hard Cases, Coding
CIF-Bench 2024-2 | Partial | ZH | HG & CI | Paper | Github | Webサイト
- Publisher: University of Manchester et al.
- Size: 15K instances
- License: -
- Question Type: SQ
- Evaluation Method: CE & ME
- Focus: Evaluate the zero-shot generalizability of LLMs to the Chinese language
- Numbers of Evaluation Categories/Subcategories: 10/150
- Evaluation Category: Chinese culture, Classification, Code, Commonsense, Creative NLG, Evaluation, Grammar, Linguistic, Motion detection, NER
WildBench 2024-6 | All | EN | HG & CI | Paper | Github | Dataset | Webサイト
- Publisher: Allen Institute for AI et al.
- Size: 1024 instances
- License: AI2 ImpACT License
- Question Type: SQ
- Evaluation Method: ME
- Focus: An automated evaluation framework designed to benchmark LLMs using challenging, real-world user queries.
- Numbers of Evaluation Categories/Subcategories: 11/-
- Evaluation Category: Information seeking, Coding & Debugging, Creative writing, Reasoning, Planning, Math, Editing, Data analysis, Role playing, Brainstorming, Advice seeking
SysBench 2024-8 | All | EN | HG | Paper | Github |データセット
- Publisher: Peking University et al.
- Size: 500 instances
- License: -
- Question Type: SQ
- Evaluation Method: ME
- Focus: Systematically analyze system message following ability
- Numbers of Evaluation Categories/Subcategories: 3/-
- Evaluation Category: Constraint complexity, Instruction misalignment, Multi-turn stability
テスト
AGIEval 2023-4 | All | EN & ZH | HG & CI | Paper | Github |データセット
- Publisher: Microsoft
- Size: 8062 instances
- License: MIT
- Question Type: OQ
- Evaluation Method: CE
- Focus: Human-centric standardized exams
- Numbers of Evaluation Categories/Subcategories: 7/20
- Evaluation Category: Gaokao, SAT, JEC, LSAT, LogiQA, AQuA-RAT, Math
GAOKAO-Bench 2023-5 | All | ZH | HG | Paper | github
- Publisher: Fudan University et al.
- Size: 2811 instances
- License: Apache-2.0
- Question Type: Multi
- Evaluation Method: HE & CE
- Focus: Chinese Gaokao examination
- Numbers of Evaluation Categories/Subcategories: 10/-
- Evaluation Category: Chinese, Mathematics (2 categories), English, Physics, Chemistry, Biology, Politics, History, Geography
M3Exam 2023-6 | All | Multi (9) | HG | Paper | github
- Publisher: Alibaba Group et al.
- Size: 12317 instances
- License: -
- Question Type: OQ
- Evaluation Method: CE
- Focus: The comprehensive abilities in a multilingual and multilevel context using real human exam questions
- Numbers of Evaluation Categories/Subcategories: 3/-
- Evaluation Category: Low, Mid, High
主題
ARB 2023-7 | All | EN | CI | Paper | github
- Publisher: DuckAI et al.
- Size: 1207 instances
- License: MIT
- Question Type: Multi
- Evaluation Method: HE & ME
- Focus: Advanced reasoning problems in multiple fields
- Numbers of Evaluation Categories/Subcategories: 5/-
- Evaluation Category: Mathematics, Physics, Law, MCAT(Reading), MCAT(Science)
C-CLUE 2021-8 | All | ZH | HG | Github | Webサイト
- Publisher: Tianjin University
- サイズ: -
- License: CC-BY-SA-4.0
- Question Type: SQ
- Evaluation Method: CE
- Focus: Classical Chinese language understanding
- Numbers of Evaluation Categories/Subcategories: 2/-
- Evaluation Category: Named entity recognition, Relation extraction
C-Eval 2023-5 | All | ZH | HG & MC | Paper | Github | Dataset | Webサイト
- Publisher: Shanghai Jiao Tong University
- Size: 13948 instances
- License: CC-BY-NC-SA-4.0
- Question Type: OQ
- Evaluation Method: CE
- Focus: The advanced knowledge and reasoning abilities in a Chinese context
- Numbers of Evaluation Categories/Subcategories: 4/52
- Evaluation Category: STEM, Social Science, Humanity, Other
CG-Eval 2023-8 | All | ZH | HG | Paper | Github | Dataset | Webサイト
- Publisher: LanguageX AI Lab et al.
- Size: 11000 instances
- License: CC-BY-SA-4.0
- Question Type: SQ
- Evaluation Method: CE
- Focus: The generation capabilities of LLMs across various academic disciplines
- Numbers of Evaluation Categories/Subcategories: 6/55
- Evaluation Category: Science and engineering, Humanities and social sciences, Mathematical calculations, Medical practitioner qualification Examination, Judicial Examination, Certfied public accountant examination
LLMEVAL-3 2023-9 | Not | ZH | HG | Github | Webサイト
- Publisher: Fudan University et al.
- Size: 200K instances
- License: -
- Question Type: SQ
- Evaluation Method: ME
- Focus: Subject-specific knowledge capability
- Numbers of Evaluation Categories/Subcategories: 13/-
- Evaluation Category: Philosophy, Economics, Law, Education, Literature, History, Science, Engineering, Agriculture, Medicine, Military science, Management, Fine arts
MMCU 2023-4 | All | ZH | HG | Paper | github
- Publisher: LanguageX AI Lab
- Size: 11845 instances
- License: -
- Question Type: OQ
- Evaluation Method: CE
- Focus: Multidisciplinary abilities
- Numbers of Evaluation Categories/Subcategories: 4/25
- Evaluation Category: Medicine, Law, Psychology, Education
MMLU 2020-9 | All | EN | HG | Paper | github
- Publisher: UC Berkeley et al.
- Size: 15908 instances
- License: MIT
- Question Type: OQ
- Evaluation Method: CE
- Focus: Knowledge in academic and professional domains
- Numbers of Evaluation Categories/Subcategories: 4/57
- Evaluation Category: Humanities, Social science, STEM, Other
M3KE 2023-5 | All | ZH | HG | Paper | Github |データセット
- Publisher: Tianjin University et al.
- Size: 20477 instances
- License: Apache-2.0
- Question Type: OQ
- Evaluation Method: CE
- Focus: Multidisciplinary abilities
- Numbers of Evaluation Categories/Subcategories: 4/71
- Evaluation Category: Arts & Humanities, Social sciences, Natural sciences, Other
SCIBENCH 2023-7 | All | EN | HG | Paper | github
- Publisher: University of California et al.
- Size: 695 instances
- License: MIT
- Question Type: SQ
- Evaluation Method: CE
- Focus: The performance in university-level science and engineering domains
- Numbers of Evaluation Categories/Subcategories: 3/10
- Evaluation Category: Physics, Chemistry, Math
ScienceQA 2022-9 | All | EN | HG | Paper | Github | Webサイト
- Publisher: University of California et al.
- Size: 21208 instances
- License: CC-BY-NC-SA-4.0
- Question Type: OQ
- Evaluation Method: CE
- Focus: Science question-answering ability
- Numbers of Evaluation Categories/Subcategories: 3/26
- Evaluation Category: Natural science, Social science, Language science
TheoremQA 2023-5 | All | EN | HG | Paper | Github |データセット
- Publisher: University of Waterloo et al.
- Size: 800 instances
- License: MIT
- Question Type: SQ
- Evaluation Method: CE
- Focus: Science subject question-answering ability
- Numbers of Evaluation Categories/Subcategories: 4/39
- Evaluation Category: Mathematics, Physics, Finance, CS & EE
XiezhiBenchmark 2023-6 | All | EN & ZH | HG & MC | Paper | github
- Publisher: Fudan University et al.
- Size: 249587 instances
- License: CC-BY-NC-SA-4.0
- Question Type: OQ
- Evaluation Method: CE
- Focus: Multidisciplinary abilities
- Numbers of Evaluation Categories/Subcategories: 13/516
- Evaluation Category: Medicine, Literature, Economics, Agronomy, Science, Jurisprudence, History, Art studies, Philosophy, Pedagogy, Military science, Management, Engineering
CMMLU 2023-6 | All | ZH | HG | Paper | Github |データセット
- Publisher: MBZUAI
- Size: 11528 instances
- License: CC-BY-NC-4.0
- Question Type: OQ
- Evaluation Method: CE
- Focus: The knowledge and reasoning capabilities within the Chinese context
- Numbers of Evaluation Categories/Subcategories: 5/67
- Evaluation Category: Social science, STEM, Humanities, China specific, Other
GPQA 2023-11 | All | EN | HG | Paper | Github |データセット
- Publisher: New York University et al.
- Size: 448 instances
- License: CC-BY-4.0
- Question Type: OQ
- Evaluation Method: CE
- Focus: The disciplinary knowledge in the fields of biology, physics, and chemistry
- Numbers of Evaluation Categories/Subcategories: 3/16
- Evaluation Category: Biology, Physics, Chemistry
CMATH 2023-6 | All | ZH | HG | Paper | Github |データセット
- Publisher: Xiaomi AI Lab
- Size: 1698 instances
- License: CC-BY-4.0
- Question Type: SQ
- Evaluation Method: CE
- Focus: Elementary school math word problems
- Numbers of Evaluation Categories/Subcategories: 6/-
- Evaluation Category: Grades 1 to 6 in elementary school
FineMath 2024-3 | Not | ZH | HG |紙
- Publisher: Tianjin University et al.
- Size: 1584 instances
- License: -
- Question Type: Multi
- Evaluation Method: -
- Focus: Elementary school math word problems
- Numbers of Evaluation Categories/Subcategories: 6/17
- Evaluation Category: Number & Operations, Measurement, Data analysis & Probability, Algebra, Geometry, Others
WYWEB 2023-7 | All | ZH | HG & CI | Paper | Github | Dataset | Webサイト
- Publisher: Zhejiang University et al.
- Size: 467200 instances
- License: -
- Question Type: Multi
- Evaluation Method: CE
- Focus: Classical Chinese
- Numbers of Evaluation Categories/Subcategories: 5/9
- Evaluation Category: Sequence labeling, Sentence classification, Token similarity, Reading comprehension, Translation
ACLUE 2023-10 | All | ZH | HG & CI | Paper | Github |データセット
- Publisher: Mohamed bin Zayed University of Artificial Intelligence
- Size: 4967 instances
- License: CC-BY-NC-SA-4.0
- Question Type: OQ
- Evaluation Method: CE
- Focus: Classical Chinese language understanding
- Numbers of Evaluation Categories/Subcategories: 5/15
- Evaluation Category: Lexical, Syntactic, Semantic, Inference, Knowledge
SciKnowEval 2024-6 | All | EN | HG & CI & MC | Paper | Github |データセット
- Publisher: Zhejiang University et al.
- Size: 50048 instances
- License: -
- Question Type: Multi
- Evaluation Method: CE & ME
- Focus: Evaluate the capabilities of LLMs in handling scientific knowledge
- Numbers of Evaluation Categories/Subcategories: 2/49
- Evaluation Category: Biology, Chemistry
C 3 Bench 2024-5 | All | ZH | HG & CI |紙
- Publisher: South China University of Technology
- Size: 50000 instances
- License: -
- Question Type: SQ
- Evaluation Method: CE
- Focus: Classical Chinese
- Numbers of Evaluation Categories/Subcategories: 5/-
- Evaluation Category: Classification, Retrieval, NER, Punctuation, Translation
ArabicMMLU 2024-8 | All | AR | HG | Paper | Github |データセット
- Publisher: MBZUAI et al.
- Size: 14575 instances
- License: CC-BY-NC-SA-4.0
- Question Type: OQ
- Evaluation Method: CE
- Focus: Multi-task language understanding benchmark for the Arabic language
- Numbers of Evaluation Categories/Subcategories: 5/40
- Evaluation Category: STEM, Social science, Humanities, Language, Other
PersianMMLU 2024-4 | All | FA | HG | Paper |データセット
- Publisher: Raia Center for Artificial Intelligence Research et al.
- Size: 20192 instances
- License: CC-ND
- Question Type: OQ
- Evaluation Method: CE
- Focus: Facilitate the rigorous evaluation of LLMs that support the Persian language
- Numbers of Evaluation Categories/Subcategories: 5/38
- Evaluation Category: Social science, Humanities, Natural science, Mathematics, Other
TMMLU+ 2024-3 | All | ZH | HG & CI | Paper |データセット
- Publisher: iKala AI Lab et al.
- Size: 22690 instances
- License: MIT
- Question Type: OQ
- Evaluation Method: CE
- Focus: Evaluate the language understanding capabilities in Traditional Chinese
- Numbers of Evaluation Categories/Subcategories: 4/66
- Evaluation Category: STEM, Social sciences, Humanities, Other
NLU
CLUE 2020-12 | All | ZH | CI | Paper | github
- Publisher: CLUE team
- Size: 9 datasets
- License: -
- Question Type: SQ
- Evaluation Method: CE
- Focus: Natural language understanding capability
- Numbers of Evaluation Categories/Subcategories: 3/9
- Evaluation Category: Single-sentence tasks, Sentence pair tasks, Machine reading comprehension tasks
CUGE 2021-12 | All | EN & ZH | CI | Paper | Webサイト
- Publisher: Tsinghua University et al.
- Size: 33.4M instances
- License: -
- Question Type: SQ
- Evaluation Method: CE
- Focus: Natural language understanding capability
- Numbers of Evaluation Categories/Subcategories: 7/18
- Evaluation Category: Language understanding (word-sentence or discourse level), Information acquisition and question answering, Language generation, Conversational interaction, Multilingual, Mathematical reasoning
GLUE 2018-11 | All | EN | CI | Paper | Github | Webサイト
- Publisher: New York University et al.
- Size: 9 datasets
- License: -
- Question Type: SQ
- Evaluation Method: CE
- Focus: Natural language understanding capability
- Numbers of Evaluation Categories/Subcategories: 3/9
- Evaluation Category: Single-sentence tasks, Similarity and paraphrase tasks, Inference tasks
SuperGLUE 2019-5 | All | EN | CI | Paper | Webサイト
- Publisher: New York University et al.
- Size: 8 datasets
- License: -
- Question Type: SQ
- Evaluation Method: CE
- Focus: Natural language understanding capability
- Numbers of Evaluation Categories/Subcategories: 4/8
- Evaluation Category: Word sense disambiguation, Natural language inference, Coreference resolution, Question answering
MCTS 2023-6 | All | ZH | HG | Paper | github
- Publisher: Beijing Language and Culture University
- Size: 723 instances
- License: -
- Question Type: SQ
- Evaluation Method: CE
- Focus: Text simplification ability
- Numbers of Evaluation Categories/Subcategories: 1/-
- Evaluation Category: Text simplification
RAFT 2021-9 | All | EN | HG & CI | Paper | Dataset | Webサイト
- Publisher: Ought et al.
- Size: 28712 instances
- License: -
- Question Type: SQ
- Evaluation Method: CE
- Focus: Text classification ability
- Numbers of Evaluation Categories/Subcategories: 1/11
- Evaluation Category: Text classification
SentEval 2018-5 | All | EN | CI | Paper | github
- Publisher: Facebook Artificial Intelligence Research
- Size: 28 datasets
- License: BSD
- Question Type: SQ
- Evaluation Method: CE
- Focus: The quality of universal sentence representations
- Numbers of Evaluation Categories/Subcategories: 1/21
- Evaluation Category: Universal sentence representations
LeSC 2024-5 | All | EN & ZH | HG | Paper | Github |データセット
- Publisher: Tsinghua University et al.
- Size: 600 instances
- License: MIT
- Question Type: OQ
- Evaluation Method: CE
- Focus: The genuine linguistic-cognitive skills of LLMs
- Numbers of Evaluation Categories/Subcategories: 1/-
- Evaluation Category: Polysemy
KoBEST 2022-10 | All | KO | CI | Paper |データセット
- Publisher: University of Oxford et al.
- Size: 5 datasets
- License: CC-BY-SA-4.0
- Question Type: OQ
- Evaluation Method: CE
- Focus: Korean balanced evaluation of significant tasks
- Numbers of Evaluation Categories/Subcategories: 5/-
- Evaluation Category: KB-BoolQ, KB-COPA, KB-WiC, KB-HellaSwag, KB-SentiNeg
SarcasmBench 2024-8 | All | EN | CI |紙
- Publisher: Tianjin University et al.
- Size: 58347 instances
- License: -
- Question Type: SQ
- Evaluation Method: CE
- Focus: Evaluate LLMs on sarcasm understanding
- Numbers of Evaluation Categories/Subcategories: 1/-
- Evaluation Category: Sarcasm understanding
Reasoning
Chain-of-Thought Hub 2023-5 | All | EN | CI | Paper | github
- Publisher: University of Edinburgh et al.
- サイズ: -
- License: MIT
- Question Type: SQ
- Evaluation Method: CE
- Focus: The multi-step reasoning capabilities
- Numbers of Evaluation Categories/Subcategories: 6/8
- Evaluation Category: Math, Science, Symbolic, Knowledge, Coding, Factual
Choice-75 2023-9 | All | EN | HG & CI & MC | Paper | github
- Publisher: University of Pittsburgh et al.
- Size: 650 instances
- License: -
- Question Type: OQ
- Evaluation Method: CE
- Focus: Predict decisions based on descriptive scenarios
- Numbers of Evaluation Categories/Subcategories: 4/-
- Evaluation Category: Easy, Medium, Hard, N/A
NeuLR 2023-6 | All | EN | CI | Paper | Github | Dataset
- Publisher: Xi'an Jiaotong University et al.
- Size: 3000 instances
- License: -
- Question Type: SQ
- Evaluation Method: CE
- Focus: Logical reasoning capabilities
- Numbers of Evaluation Categories/Subcategories: 3/-
- Evaluation Category: Deductive, Inductive, Abductive
TabMWP 2022-9 | All | EN | HG | Paper | Github | Webサイト
- Publisher: University of California et al.
- Size: 38431 instances
- License: CC-BY-NC-SA-4.0
- Question Type: Multi
- Evaluation Method: CE
- Focus: Mathematical reasoning ability involving both textual and tabular information
- Numbers of Evaluation Categories/Subcategories: 1/-
- Evaluation Category: Mathematical reasoning and table QA
LILA 2022-10 | All | EN | CI | Paper | Github | Dataset
- Publisher: Arizona State Univeristy et al.
- Size: 317262 instances
- License: CC-BY-4.0
- Question Type: Multi
- Evaluation Method: CE
- Focus: Mathematical reasoning across diverse tasks
- Numbers of Evaluation Categories/Subcategories: 4/23
- Evaluation Category: Math ability, Language, Knowledge, Format
MiniF2F_v1 2021-9 | All | EN | HG & CI | Paper | github
- Publisher: Ecole Polytechnique et al.
- Size: 488 instances
- License: -
- Question Type: SQ
- Evaluation Method: CE
- Focus: The performance on formal Olympiad-level mathematics problem statements
- Numbers of Evaluation Categories/Subcategories: 1/-
- Evaluation Category: Math
GameBench 2024-6 | All | EN | HG | Paper | Github | Dataset
- Publisher: Olin College of Engineering et al.
- Size: 9 Games
- License: CC-BY
- Question Type: SQ
- Evaluation Method: CE
- Focus: Evaluate strategic reasoning abilities of LLM agents
- Numbers of Evaluation Categories/Subcategories: 6/9
- Evaluation Category: Abstract Strategy, Non-Deterministic, Hidden Information, Language Communication, Social Deduction, Cooperation
TableBench 2024-8 | All | EN | HG & CI & MC | Paper | Github | Dataset | Webサイト
- Publisher: Beihang University et al.
- Size: 886 instances
- License: Apache-2.0
- Question Type: SQ
- Evaluation Method: CE
- Focus: Table question answering (TableQA) capabilities
- Numbers of Evaluation Categories/Subcategories: 4/18
- Evaluation Category: Fact checking, Numerical reasoning, Data analysis, Visualization
知識
ALCUNA 2023-10 | All | EN | HG | Paper | Github | Dataset
- Publisher: Peking University
- Size: 84351 instances
- License: MIT
- Question Type: Multi
- Evaluation Method: CE
- Focus: Assess the ability of LLMs to respond to new knowledge
- Numbers of Evaluation Categories/Subcategories: 3/-
- Evaluation Category: Knowledge understanding, Knowledge differentiation, Knowledge association
KoLA 2023-6 | Partial | EN | HG & CI | Paper | Github | Webサイト
- Publisher: Tsinghua University
- Size: 2138 instances
- License: GPL-3.0
- Question Type: SQ
- Evaluation Method: CE
- Focus: The ability to grasp and utilize world knowledge
- Numbers of Evaluation Categories/Subcategories: 4/19
- Evaluation Category: Knowledge memorization, Knowledge understanding, Knowledge applying, Knowledge creating
LLMEVAL-2 2023-7 | All | ZH | HG | github
- Publisher: Fudan University et al.
- Size: 480 instances
- License: -
- Question Type: Multi
- Evaluation Method: HE & ME
- Focus: Knowledge capability
- Numbers of Evaluation Categories/Subcategories: 12/-
- Evaluation Category: Computer science, Economics, Foreign languages, Law, Mathematics, Medicine, Optics, Physics, Social sciences, Chinese language and literature, Chemistry, Life sciences
SocKET 2023-5 | All | EN | CI | Paper | github
- Publisher: University of Michigan et al.
- Size: 2616342 instances
- License: CC-BY-4.0
- Question Type: SQ
- Evaluation Method: CE
- Focus: Mastery of social knowledge
- Numbers of Evaluation Categories/Subcategories: 4/58
- Evaluation Category: Classification, Regression, Pair-wise comparison, Span identification
LMExamQA 2023-6 | All | EN | MC | Paper | Webサイト
- Publisher: Tsinghua University et al.
- Size: 10090 instances
- License: -
- Question Type: SQ
- Evaluation Method: ME
- Focus: The performance on open-ended question answering
- Numbers of Evaluation Categories/Subcategories: 3/25
- Evaluation Category: Knowledge memorization, Knowledge comprehension, Knowledge analysis
DebateQA 2024-8 | All | EN | HG & CI & MC | Paper | Github | Dataset
- Publisher: Tsinghua Universty et al.
- Size: 2941 instances
- License: -
- Question Type: SQ
- Evaluation Method: ME
- Focus: Evaluate the comprehensiveness of perspectives and assess whether the LLM acknowledges the question's debatable nature
- Numbers of Evaluation Categories/Subcategories: 2/-
- Evaluation Category: Perspective diversity, Dispute awareness
Long Text
L-Eval 2023-7 | All | EN | HG & CI | Paper | Github | Dataset
- Publisher: Fudan University et al.
- Size: 2043 instances
- License: GPL-3.0
- Question Type: SQ
- Evaluation Method: HE & CE & ME
- Focus: Long text task capability
- Numbers of Evaluation Categories/Subcategories: 1/18
- Evaluation Category: Long text task
LongBench 2023-8 | All | EN & ZH | CI | Paper | Github | Dataset
- Publisher: Tsinghua University et al.
- Size: 4750 instances
- License: MIT
- Question Type: SQ
- Evaluation Method: CE
- Focus: Long text task capability
- Numbers of Evaluation Categories/Subcategories: 6/21
- Evaluation Category: Single-doc QA, Multi-doc QA, Summarization, Few-shot learning, Synthetic tasks, Code completion
LongEval 2023-6 | All | EN | HG | Github | Webサイト
- Publisher: LMSYS
- サイズ: -
- License: Apache-2.0
- Question Type: SQ
- Evaluation Method: CE
- Focus: Long text task capability
- Numbers of Evaluation Categories/Subcategories: 2/-
- Evaluation Category: Coarse-grained topic retrieval, Fine-grained line retrieval
InfiniteBench 2023-11 | All | EN & ZH | HG & CI & MC | Github | Dataset
- Publisher: Tsinghua University et al.
- Size: 3932 instances
- License: Apache-2.0
- Question Type: Multi
- Evaluation Method: -
- Focus: Long text task capability
- Numbers of Evaluation Categories/Subcategories: 5/12
- Evaluation Category: Mathematics, Code, Dialogue, Books, Retrieval
ZeroSCROLLS 2023-5 | All | EN | HG & CI | Paper | Github | Dataset | Webサイト
- Publisher: Tel Aviv University et al.
- Size: 4378 instances
- License: MIT
- Question Type: Multi
- Evaluation Method: CE
- Focus: Long text task capability
- Numbers of Evaluation Categories/Subcategories: 3/10
- Evaluation Category: Summarization, Question Answering, Aggregation
LooGLE 2023-11 | All | EN | HG & CI & MC | Paper | Github | Dataset
- Publisher: BIGAI et al.
- Size: 6448 instances
- License: CC-BY-SA-4.0
- Question Type: SQ
- Evaluation Method: HE & CE & ME
- Focus: Long text task capability
- Numbers of Evaluation Categories/Subcategories: 2/4
- Evaluation Category: Long dependency tasks, Short dependency tasks
NAH (Needle-in-a-Haystack) 2023-11 | All | EN | - | github
- Publisher: gkamradt et al.
- サイズ: -
- License: MIT
- Question Type: SQ
- Evaluation Method: ME
- Focus: Long text task capability
- Numbers of Evaluation Categories/Subcategories: 1/-
- Evaluation Category: Long text task
CLongEval 2024-3 | All | ZH | HG & CI & MC | Paper | Github | Dataset
- Publisher: The Chinese University of Hong Kong et al.
- Size: 7267 instances
- License: MIT
- Question Type: SQ
- Evaluation Method: CE
- Focus: Long text task capability
- Numbers of Evaluation Categories/Subcategories: 7/-
- Evaluation Category: Long story QA, Long conversation memory, Long story summarization, Stacked news labeling, Stacked typo detection, Key-passage retrieval, Table querying
Counting-Stars 2024-3 | All | ZH | HG | Paper | Github | Dataset
- Publisher: Tencent MLPD
- サイズ: -
- License: -
- Question Type: SQ
- Evaluation Method: CE
- Focus: Long text task capability
- Numbers of Evaluation Categories/Subcategories: 1/-
- Evaluation Category: Long text task
NeedleBench 2024-7 | All | EN & ZH | HG & CI | Paper | github
- Publisher: Shanghai AI Laboratory et al.
- サイズ: -
- License: -
- Question Type: Multi
- Evaluation Method: CE
- Focus: Assess bilingual long-context capabilities
- Numbers of Evaluation Categories/Subcategories: 3/-
- Evaluation Category: Single-retrieval, Multi-retrieval, Multi-reasoning
道具
API-Bank 2023-4 | All | EN & PL | HG & MC | Paper | github
- Publisher: Alibaba DAMO Academy et al.
- Size: 264 dialogues
- License: MIT
- Question Type: SQ
- Evaluation Method: HE & CE
- Focus: Plan step-by-step API calls, retrieve relevant APIs, and correctly execute API calls to meet human needs
- Numbers of Evaluation Categories/Subcategories: 3/-
- Evaluation Category: Call, Retrieval+Call, Plan+Retrieval+Call
APIBench 2023-5 | All | EN & PL | HG & MC | Paper | Github | Dataset | Webサイト
- Publisher: UC Berkeley et al.
- Size: 16450 instances
- License: Apache-2.0
- Question Type: SQ
- Evaluation Method: CE
- Focus: The reasoning ability for calling APIs
- Numbers of Evaluation Categories/Subcategories: 1/-
- Evaluation Category: API call
ToolBench 2023-5 | All | EN | HG & CI | Paper | github
- Publisher: SambaNova Systems et al.
- Size: 795 instances
- License: Apache-2.0
- Question Type: SQ
- Evaluation Method: CE
- Focus: The enhancement in tool manipulation for real-world software tasks
- Numbers of Evaluation Categories/Subcategories: 8/-
- Evaluation Category: Open weather, The cat API, Home search, Trip booking, Google sheets, Virtual home, Web shop, Tabletop
ToolEyes 2024-1 | All | EN | HG | Paper | Github |データセット
- Publisher: Fudan University
- Size: 382 instances
- License: Apache-2.0
- Question Type: SQ
- Evaluation Method: CE & ME
- Focus: The LLMs' tool learning capabilities in authentic scenarios
- Numbers of Evaluation Categories/Subcategories: 7/41
- Evaluation Category: Text generation, Data understanding, Real-time search, Application manipulation, Personal life, Information retrieval, Financial transactions
エージェント
コード
BIRD 2023-5 | All | EN & PL | HG & CI & MC | Paper | Github | Dataset | Webサイト
- Publisher: The University of Hong Kong et al.
- Size: 12751 instances
- License: CC-BY-NC-4.0
- Question Type: SQ
- Evaluation Method: CE
- Focus: Text-to-SQL parsing
- Numbers of Evaluation Categories/Subcategories: 1/-
- Evaluation Category: Text-SQL
CodeXGLUE 2021-2 | All | EN & PL | CI | Paper | Github | Dataset
- Publisher: Peking University et al.
- Size: 4.12M instances
- License: C-UDA
- Question Type: SQ
- Evaluation Method: CE
- Focus: Program understanding and generation tasks
- Numbers of Evaluation Categories/Subcategories: 4/10
- Evaluation Category: Code-Code, Text-Code, Code-Text, Text-to-Text
DS-1000 2022-11 | All | EN & PL | HG | Paper | Github | Dataset | Webサイト
- Publisher: The University of Hong Kong et al.
- Size: 1000 instances
- License: CC-BY-SA-4.0
- Question Type: SQ
- Evaluation Method: CE
- Focus: Code generation
- Numbers of Evaluation Categories/Subcategories: 1/-
- Evaluation Category: Code generation
HumanEval 2021-7 | All | EN & PL | HG | Paper | github
- Publisher: OpenAI et al.
- Size: 164 instances
- License: MIT
- Question Type: SQ
- Evaluation Method: CE
- Focus: The correctness of problem-solving abilities in the context of program synthesis
- Numbers of Evaluation Categories/Subcategories: 1/-
- Evaluation Category: Code generation
HumanEvalPack 2023-8 | All | EN & PL | HG & CI | Paper | Github | Dataset
- Publisher: Bigcode
- Size: 984 instances
- License: MIT
- Question Type: SQ
- Evaluation Method: CE
- Focus: The correctness of problem-solving abilities in the context of program synthesis
- Numbers of Evaluation Categories/Subcategories: 3/-
- Evaluation Category: HumanEvalFix, HumanEvalExplain, HumanEvalSynthesize
MTPB 2022-3 | All | EN & PL | HG | Paper | Github | Dataset
- Publisher: Salesforce Research
- Size: 115 instances
- License: Apache-2.0
- Question Type: SQ
- Evaluation Method: CE
- Focus: Multi-turn Programming
- Numbers of Evaluation Categories/Subcategories: 1/-
- Evaluation Category: Code generation
ODEX 2022-12 | All | Multi & PL | HG & CI | Paper | github
- Publisher: Carnegie Mellon University et al.
- Size: 945 instances
- License: CC-BY-SA-4.0
- Question Type: SQ
- Evaluation Method: CE
- Focus: Natural language to Python code generation
- Numbers of Evaluation Categories/Subcategories: 1/-
- Evaluation Category: Code generation
APPS 2021-5 | All | EN & PL | HG | Paper | Github | Dataset
- Publisher: UC Berkeley et al.
- Size: 10000 instances
- License: MIT
- Question Type: SQ
- Evaluation Method: CE
- Focus: The ability to take an arbitrary natural language specification and generate satisfactory Python code
- Numbers of Evaluation Categories/Subcategories: 1/-
- Evaluation Category: Code generation
DomainEval 2024-8 | All | EN & PL | HG & CI & MC | Paper | Github | Dataset | Webサイト
- Publisher: Chinese Academy of Sciences et al.
- Size: 5892 instances
- License: -
- Question Type: SQ
- Evaluation Method: CE
- Focus: Evaluate LLMs' coding capabilities thoroughly
- Numbers of Evaluation Categories/Subcategories: 6/-
- Evaluation Category: Computation, Network, Basic operation, System, Visualization, Cryptography
OOD
法
LAiW 2023-10 | Partial | ZH | CI | Paper | github
- Publisher: Sichuan University et al.
- サイズ: -
- License: -
- Question Type: SQ
- Evaluation Method: CE
- Focus: Legal capabilities
- Numbers of Evaluation Categories/Subcategories: 3/13
- Evaluation Category: Basic legal NLP, Basic legal application, Complex legal application
LawBench 2023-9 | All | ZH | HG & CI | Paper | Github | Dataset
- Publisher: Nanjing University et al.
- サイズ: -
- License: Apache-2.0
- Question Type: Multi
- Evaluation Method: CE
- Focus: Legal capabilities
- Numbers of Evaluation Categories/Subcategories: 3/20
- Evaluation Category: Legal knowledge memorization, Legal knowledge understanding, Legal knowledge applying
LegalBench 2023-8 | All | EN | HG & CI | Paper | Github | Dataset | Webサイト
- Publisher: Stanford University et al.
- Size: 90417 instances
- License: -
- Question Type: SQ
- Evaluation Method: HE & CE
- Focus: Legal reasoning
- Numbers of Evaluation Categories/Subcategories: 6/162
- Evaluation Category: Issue-spotting, Rule-recall, Rule-application, Rule-conclusion, Interpretation, Rhetorical-understanding
LexGLUE 2021-10 | All | EN | CI | Paper | github
- Publisher: University of Copenhagen et al.
- Size: 237014 instances
- License: -
- Question Type: SQ
- Evaluation Method: CE
- Focus: Legal capabilities
- Numbers of Evaluation Categories/Subcategories: 3/-
- Evaluation Category: Multi-label classification, Multi-class classification, Multiple choice QA
LEXTREME 2023-1 | All | Multi (24) | CI | Paper | github
- Publisher: University of Bern et al.
- Size: 3508603 instances
- License: -
- Question Type: SQ
- Evaluation Method: CE
- Focus: Legal capabilities
- Numbers of Evaluation Categories/Subcategories: 18/-
- Evaluation Category: Brazilian court decisions, German argument mining, Greek legal code, Swiss judgment prediction, etc.
SCALE 2023-6 | All | Multi (5) | HG & CI | Paper | Dataset
- Publisher: University of Bern et al.
- Size: 1.86M instances
- License: CC-BY-SA
- Question Type: SQ
- Evaluation Method: CE
- Focus: Legal multidimensional abilities
- Numbers of Evaluation Categories/Subcategories: 4/-
- Evaluation Category: Processing long documents, Utilizing domain specific knowledge, Multilingual understanding, Multitasking
ArabLegalEval 2024-8 | All | AR | HG & CI & MC | Paper | Github | Dataset
- Publisher: THIQAH et al.
- Size: 37853 instances
- License: -
- Question Type: Multi
- Evaluation Method: ME
- Focus: Assess the Arabic legal knowledge of LLMs
- Numbers of Evaluation Categories/Subcategories: 3/-
医学
CBLUE 2022-5 | All | ZH | HG & CI | Paper | github
- Publisher: Zhejiang University et al.
- Size: 195820 instances
- License: Apache-2.0
- Question Type: SQ
- Evaluation Method: CE
- Focus: Chinese biomedical language understanding
- Numbers of Evaluation Categories/Subcategories: 5/8
- Evaluation Category: Information extraction from the medical text, normalization of the medical term, medical text classification, medical sentence similarity estimation, medical QA
CMB 2023-8 | All | ZH | HG | Paper | Github | Dataset | Webサイト
- Publisher: The Chinese University of Hong Kong et al.
- Size: 281047 instances
- License: Apache-2.0
- Question Type: Multi
- Evaluation Method: HE & CE & ME
- Focus: The performance of LLMs in the field of medicine
- Numbers of Evaluation Categories/Subcategories: 2/7
- Evaluation Category: CMB-Exam, CMB-Clin
HuaTuo26M-test 2023-5 | All | ZH | CI | Paper | Github | Dataset
- Publisher: The Chinese University of Hong Kong et al.
- Size: 6000 instances
- License: Apache-2.0
- Question Type: SQ
- Evaluation Method: CE
- Focus: Understand and generate complex medical language
- Numbers of Evaluation Categories/Subcategories: 3/-
- Evaluation Category: Medical consultant records, Encyclopedias, Knowledge bases
MultiMedQA 2022-12 | All | EN | HG & CI | Paper | Dataset
- Publisher: Google Research et al.
- Size: 212822 instances
- License: -
- Question Type: Multi
- Evaluation Method: HE & CE
- Focus: The performance in medical and clinical applications
- Numbers of Evaluation Categories/Subcategories: 1/-
- Evaluation Category: Medical question answering
PromptCBLUE 2023-4 | All | ZH | CI | github
- Publisher: East China Normal University et al.
- Size: 20640 instances
- License: -
- Question Type: SQ
- Evaluation Method: CE
- Focus: The performance in Chinese medical scenarios
- Numbers of Evaluation Categories/Subcategories: 16/-
- Evaluation Category: Medical named entity recognition, Medical entity relation extraction, Medical event extraction, etc.
QiZhenGPT_eval 2023-5 | All | ZH | HG | Github | Dataset
- Publisher: Zhejiang University et al.
- Size: 94 instances
- License: GPL-3.0
- Question Type: SQ
- Evaluation Method: HE
- Focus: Indications for use of drugs
- Numbers of Evaluation Categories/Subcategories: 1/-
- Evaluation Category: Drug indication question answering
CLUE 2024-4 | Partical | EN | HG & CI & MC | Paper | github
- Publisher: University Hospital Essen et al.
- サイズ: -
- License: -
- Question Type: SQ
- Evaluation Method: CE
- Focus: Real-world clinical tasks
- Numbers of Evaluation Categories/Subcategories: 6/-
- Evaluation Category: MeDiSumQA, MeDiSumCode, MedNLI, MeQSum, Problem Summary, LongHealth
金融
BBF-CFLEB 2023-2 | All | ZH | HG & CI | Paper | Github | Webサイト
- Publisher: Fudan University et al.
- Size: 11327 instances
- License: -
- Question Type: SQ
- Evaluation Method: CE
- Focus: Language understanding and generation tasks in Chinese financial natural language processing
- Numbers of Evaluation Categories/Subcategories: 6/-
- Evaluation Category: FinNL, FinNA, FinRE, FinFE, FinQA, FinNSP
FinancelQ 2023-9 | All | ZH | HG & MC | github
- Publisher: Du Xiaoman
- Size: 7173 instances
- License: CC-BY-NC-SA-4.0
- Question Type: OQ
- Evaluation Method: CE
- Focus: The knowledge and reasoning abilities in financial contexts
- Numbers of Evaluation Categories/Subcategories: 10/36
- Evaluation Category: Bank, Fund, Securities, Futures and derivatives, CICE, Actuarial science, Financial planning, CPA, Taxation, Economics
FinEval 2023-8 | All | ZH | HG | Paper | Github | Dataset | Webサイト
- Publisher: Shanghai University of Finance and Economics
- Size: 4661 instances
- License: CC-BY-NC-SA-4.0
- Question Type: OQ
- Evaluation Method: CE
- Focus: The performance in the financial domain knowledge
- Numbers of Evaluation Categories/Subcategories: 4/34
- Evaluation Category: Finance, Economy, Accounting, Certificate
FLUE 2022-10 | All | EN | CI | Paper | Webサイト
- Publisher: Georgia Institute of Technology et al.
- Size: 26292 instances
- License: -
- Question Type: SQ
- Evaluation Method: CE
- Focus: NLP tasks in the financial domain
- Numbers of Evaluation Categories/Subcategories: 5/6
- Evaluation Category: Financial sentiment analysis, News headline classification, Named entity recognition, Structure boundary detection, Question answering
FinBen 2024-2 | All | EN | CI | Paper | github
- Publisher: The Fin AI et al.
- Size: 69805 instances
- License: -
- Question Type: SQ
- Evaluation Method: CE
- Focus: NLP tasks in the financial domain
- Numbers of Evaluation Categories/Subcategories: 3/6
- Evaluation Category: Foundamental tasks, Advanced cognitive engagement, General intelligence
Social Norms
CrowS-Pairs 2020-11 | All | EN | HG & CI | Paper | github
- Publisher: New York University
- Size: 1508 instances
- License: CC-SA-4.0
- Question Type: SQ
- Evaluation Method: CE
- Focus: The presence of cultural biases and stereotypes in pretrained language models
- Numbers of Evaluation Categories/Subcategories: 9/-
- Evaluation Category: Race, Gender, Sexual orientation, Religion, Age, Nationality, Disability, Physical appearance, Occupation
SafetyBench 2023-9 | All | EN & ZH | HG & CI & MC | Paper | Github | Dataset | Webサイト
- Publisher: Tsinghua University et al.
- Size: 11435 instances
- License: MIT
- Question Type: OQ
- Evaluation Method: CE
- Focus: The safety of LLMs
- Numbers of Evaluation Categories/Subcategories: 7/-
- Evaluation Category: Offensiveness, Unfairness and bias, Physical health, Mental Health, Illegal activities, Ethics and morality, Privacy and Property
Safety-Prompts 2023-4 | Partial | ZH | MC | Paper | Github | Dataset | Webサイト
- Publisher: Tsinghua University et al.
- Size: 100K instances
- License: Apache-2.0
- Question Type: SQ
- Evaluation Method: HE & ME
- Focus: The safety of LLMs
- Numbers of Evaluation Categories/Subcategories: 2/13
- Evaluation Category: Typical security scenarios, Instruction attack
SuperCLUE-Safety 2023-9 | Not | ZH | - | Github | Webサイト
- Publisher: CLUEbenchmark
- Size: 4912 instances
- License: -
- Question Type: SQ
- Evaluation Method: ME
- Focus: The safety of LLMs
- Numbers of Evaluation Categories/Subcategories: 3/20+
- Evaluation Category: Traditional security category, Responsible artificial intelligence, Instruction attacks
TRUSTGPT 2023-6 | All | EN | CI | Paper | github
- Publisher: Sichuan University et al.
- Size: 2000 instances
- License: MIT
- Question Type: SQ
- Evaluation Method: CE
- Focus: The performance in toxicity, bias, and value alignment
- Numbers of Evaluation Categories/Subcategories: 3/-
- Evaluation Category: Toxicity, Bias, Value-alignment
Factuality
FACTOR 2023-7 | Partial | EN | HG & CI & MC | Paper | github
- Publisher: AI21 Labs
- Size: 4030 instances
- License: MIT
- Question Type: OQ
- Evaluation Method: CE
- Focus: The factuality of LLMs
- Numbers of Evaluation Categories/Subcategories: 2/-
- Evaluation Category: Wiki, News
FActScore 2023-5 | All | EN | HG & MC | Paper | github
- Publisher: University of Washington et al.
- Size: 500 instances
- License: MIT
- Question Type: SQ
- Evaluation Method: HE & ME
- Focus: The factuality of LLMs
- Numbers of Evaluation Categories/Subcategories: 7/-
- Evaluation Category: Single-sentence contradiction (words or beyond words), Page-level contradiction, Subjective, Fact is irrelevant, Wiki is inconsistent & wrong, Annotation error
FactualityPrompt 2022-6 | All | EN | CI | Paper | github
- Publisher: Hong Kong University of Science and Technology et al.
- Size: 16000 instances
- License: Apache-2.0
- Question Type: SQ
- Evaluation Method: CE
- Focus: The factuality of LLMs
- Numbers of Evaluation Categories/Subcategories: 2/-
- Evaluation Category: Factual prompts, Nonfactual prompts
FreshQA 2023-10 | All | EN | HG | Paper | github
- Publisher: Google et al.
- Size: 600 instances
- License: -
- Question Type: SQ
- Evaluation Method: HE
- Focus: The factuality of LLMs
- Numbers of Evaluation Categories/Subcategories: 4/-
- Evaluation Category: Never-changing, Slow-changing, Fast-changing, False-premise
HalluQA 2023-10 | All | ZH | HG & MC | Paper | github
- Publisher: Fudan University et al.
- Size: 450 instances
- License: -
- Question Type: SQ
- Evaluation Method: ME
- Focus: The factuality of LLMs
- Numbers of Evaluation Categories/Subcategories: 3/-
- Evaluation Category: Misleading, Misleading-hard, Knowledge
HaluEval 2023-5 | All | EN | HG & CI & MC | Paper | Github | Dataset
- Publisher: Renmin University of China et al.
- Size: 35000 instances
- License: MIT
- Question Type: SQ
- Evaluation Method: CE
- Focus: The factuality of LLMs
- Numbers of Evaluation Categories/Subcategories: 3/-
- Evaluation Category: QA, Dialogue, Summarization
TruthfulQA 2022-5 | All | EN | HG | Paper | github
- Publisher: University of Oxford et al.
- Size: 817 instances
- License: Apache-2.0
- Question Type: SQ
- Evaluation Method: CE & ME
- Focus: The factuality of LLMs
- Numbers of Evaluation Categories/Subcategories: 38/-
- Evaluation Category: Health, Law, Conspiracies, Fiction, Misconceptions, Paranormal, Economics, Biology, Language, Indexical etc.
UHGEval 2023-11 | All | ZH | HG & MC | Paper | Github | Dataset
- Publisher: Renmin University of China et al.
- Size: 5141 instances
- License: Apache-2.0
- Question Type: Multi
- Evaluation Method: CE
- Focus: The factuality of LLMs
- Numbers of Evaluation Categories/Subcategories: 3/4
- Evaluation Category: Discriminative, Selective, Generative
HaluEval-Wild 2024-3 | Not | EN | HG & CI & MC |紙
- Publisher: Carnegie Mellon University
- Size: 500 instances
- License: -
- Question Type: SQ
- Evaluation Method: -
- Focus: The factuality of LLMs
- Numbers of Evaluation Categories/Subcategories: 5/-
- Evaluation Category: Out-of-scope information, Complex reasoning, Inappropriate content, Beyond-modality interaction, Confused / Erroneous queries
RealTime QA 2022-7 | All | EN | HG | Paper | Github | Dataset | Webサイト
- Publisher: Toyota Technological Institute et al.
- サイズ: -
- License: -
- Question Type: Multi
- Evaluation Method: CE
- Focus: The factuality of LLMs
- Numbers of Evaluation Categories/Subcategories: 1/-
- Evaluation Category: Latest knowledge Q&A
ChineseFactEval 2023-9 | All | ZH | HG & MC | Github | Dataset | Webサイト
- Publisher: Shanghai Jiao Tong University et al.
- Size: 125 instances
- License: Apache-2.0
- Question Type: SQ
- Evaluation Method: -
- Focus: The factuality of LLMs
- Numbers of Evaluation Categories/Subcategories: 7/-
- Evaluation Category: General domain, Scientific research, Medical, Law, Finance, Math, Chinese modern history
HalluDial 2024-6 | All | EN | CI & MC | Paper | Github | Dataset
- Publisher: BAAI et al.
- Size: 146856 instances
- License: -
- Question Type: SQ
- Evaluation Method: HE & CE & ME
- Focus: Automatic dialogue-level hallucination evaluation
- Numbers of Evaluation Categories/Subcategories: 2/-
- Evaluation Category: Hallucination detection, Hallucination localization and explanation
評価
FairEval 2023-5 | All | EN | CI | Paper | Github | Dataset
- Publisher: Peking University et al.
- Size: 80 instances
- License: -
- Question Type: SQ
- Evaluation Method: CE
- Focus: The performance in determining the quality of output content from different models
- Numbers of Evaluation Categories/Subcategories: 1/-
- Evaluation Category: Evaluate the quality of answers
LLMEval2 2023-8 | All | Multi | CI | Paper | Github | Dataset
- Publisher: Chinese Academy of Sciences et al.
- Size: 2533 instances
- License: MIT
- Question Type: SQ
- Evaluation Method: CE
- Focus: The performance in determining the quality of output content from different models
- Numbers of Evaluation Categories/Subcategories: 1/-
- Evaluation Category: Evaluate the quality of answers
PandaLM_testset 2023-4 | All | EN | HG & MC | Paper | github
- Publisher: Peking University et al.
- Size: 999 instances
- License: Apache-2.0
- Question Type: SQ
- Evaluation Method: CE
- Focus: The performance in determining the quality of output content from different models
- Numbers of Evaluation Categories/Subcategories: 1/-
- Evaluation Category: Evaluate the quality of answers
Multitask
BBH 2022-10 | All | EN | CI | Paper | github
- Publisher: Google Research et al.
- Size: 6511 instances
- License: MIT
- Question Type: Multi
- Evaluation Method: CE
- Focus: Challenging tasks that have proven difficult for prior language model evaluations
- Numbers of Evaluation Categories/Subcategories: 23/27
- Evaluation Category: Boolean expressions, Causal judgement, Date understanding, Disambiguation QA, etc.
BIG-Bench 2022-6 | All | Multi | HG & CI | Paper | github
- Publisher: Google et al.
- サイズ: -
- License: Apache-2.0
- Question Type: Multi
- Evaluation Method: CE
- Focus: The capabilities and limitations of language models
- Numbers of Evaluation Categories/Subcategories: 95/204
- Evaluation Category: Linguistics, Child development, Mathematics, Common sense reasoning, Biology, etc.
CLEVA 2023-8 | All | ZH | HG & CI | Paper | Github | Webサイト
- Publisher: The Chinese University of Hong Kong et al.
- Size: 370K instances
- License: CC-BY-NC-ND-4.0
- Question Type: SQ
- Evaluation Method: CE
- Focus: The performance of LLMs across various dimensions
- Numbers of Evaluation Categories/Subcategories: 2/31
- Evaluation Category: Ability, Application
CLiB 2023-6 | All | ZH | - | github
- Publisher: jeinlee1991
- Size: 90 instances
- License: -
- Question Type: SQ
- Evaluation Method: HE
- Focus: Multidimensional capabilities
- Numbers of Evaluation Categories/Subcategories: 4/-
- Evaluation Category: Classification, Information extraction, Reading comprehension, Tabular question answering
decaNLP 2018-6 | All | EN | CI | Paper | github
- Publisher: Salesforce Research
- Size: 2010693 instances
- License: BSD-3-Clause
- Question Type: SQ
- Evaluation Method: CE
- Focus: Multitask natural language processing capabilities
- Numbers of Evaluation Categories/Subcategories: 10/-
- Evaluation Category: Question answering, Machine translaion, Summarization, Natural language inference, Sentiment analysis, Semantic role labeling, Zero-shot relation extraction, Goal-oriented dialogue, Semantic parsing, Pronoun resolution
FlagEval 2023-6 | Partial | EN & ZH | HG & CI | Github | Webサイト
- Publisher: BAAI et al.
- Size: 84433 instances
- License: -
- Question Type: Multi
- Evaluation Method: HE & CE
- Focus: Multi-domain, multi-dimensional capabilities
- Numbers of Evaluation Categories/Subcategories: 3/21
- Evaluation Category: Choice qa, Classification, Generation qa
HELM 2022-11 | All | EN | CI | Paper | Github | Webサイト
- Publisher: Stanford University et al.
- サイズ: -
- License: Apache-2.0
- Question Type: SQ
- Evaluation Method: HE & CE
- Focus: Evaluate LLMs on a wide range of scenarios and metrics
- Numbers of Evaluation Categories/Subcategories: 73/-
- Evaluation Category: Question answering, Information retrieval, Sentiment analysis, Toxicity detection, Aspirational scenarios, etc.
LLMEVAL-1 2023-5 | All | ZH | HG | github
- Publisher: Fudan University et al.
- Size: 453 instances
- License: -
- Question Type: SQ
- Evaluation Method: HE & ME
- Focus: Multidimensional capabilities
- Numbers of Evaluation Categories/Subcategories: 17/-
- Evaluation Category: Fact-based question answering, Reading comprehension, Framework generation, Paragraph rewriting, etc.
LMentry 2023-7 | All | EN | HG | Paper | github
- Publisher: Tel Aviv University et al.
- Size: 110703 instances
- License: -
- Question Type: SQ
- Evaluation Method: CE
- Focus: The performance on challenging tasks
- Numbers of Evaluation Categories/Subcategories: 25/-
- Evaluation Category: Sentence containing word, Sentence not containing word, Word containing letter, Word not containing letter, etc.
AlignBench 2023-11 | All | ZH | HG & MC | Paper | Github | Dataset
- Publisher: Tsinghua University et al.
- Size: 683 instances
- License: -
- Question Type: Multi
- Evaluation Method: ME
- Focus: Evaluate the alignment of LLMs on Chinese multitasks.
- Numbers of Evaluation Categories/Subcategories: 8/-
- Evaluation Category: Fundamental language ability, Advanced Chinese understanding, Open-ended questions, Writing ability, Logical reasoning, Mathematics, Task-oriented role play,
- Professional knowledge
多言語
XNLI 2018-10 | All | Multi (15) | HG | Paper | github
- Publisher: Facebook AI et al.
- Size: 112500 instances
- License: CC-BY-NC-4.0
- Question Type: SQ
- Evaluation Method: CE
- Focus: Multilingual NLI
- Numbers of Evaluation Categories/Subcategories: 1/-
- Evaluation Category: Multilingual natural language inference
XTREME 2020-3 | All | Multi (40) | CI | Paper | Github | Webサイト
- Publisher: Carnegie Mellon University et al.
- サイズ: -
- License: Apache-2.0
- Question Type: SQ
- Evaluation Method: CE
- Focus: The cross-lingual generalization capabilities
- Numbers of Evaluation Categories/Subcategories: 4/9
- Evaluation Category: Classification, Structured prediction, QA, Retrieval
MGSM 2022-10 | All | Multi (10) | CI | Paper | Github | Dataset
- Publisher: Google Research et al.
- Size: 2580 instances
- License: CC-BY-SA-4.0
- Question Type: SQ
- Evaluation Method: CE
- Focus: Multilingual mathematical reasoning abilities
- Numbers of Evaluation Categories/Subcategories: 1/-
- Evaluation Category: Math
他の
EcomGPT_eval 2023-8 | All | EN & ZH | CI | Paper | github
- Publisher: Alibaba
- Size: 6000 instances
- License: -
- Question Type: SQ
- Evaluation Method: CE
- Focus: E-commerce-related tasks
- Numbers of Evaluation Categories/Subcategories: 4/12
- Evaluation Category: Classification, Generation, Extraction, Others
- Domain: E-commerce
FewCLUE 2021-7 | Partial | ZH | CI | Paper | Github | Webサイト
- Publisher: CLUE team
- Size: 9 datasets
- License: -
- Question Type: SQ
- Evaluation Method: CE
- Focus: Compare different few-shot learning methods
- Numbers of Evaluation Categories/Subcategories: 3/9
- Evaluation Category: Single sentence tasks, Sentence pair tasks, Reading comprehension
- Domain: Few-shot learning
GeoBench 2023-6 | All | EN | HG | Paper | github
- Publisher: Shanghai Jiao Tong University et al.
- Size: 2517 instances
- License: Apache-2.0
- Question Type: Multi
- Evaluation Method: HE & CE & ME
- Focus: LLMs' performance in understanding and utilizing geoscience knowledge
- Numbers of Evaluation Categories/Subcategories: 2/-
- Evaluation Category: NPEE, APTest
- Domain: Geoscience
Owl-Bench 2023-9 | All | EN & ZH | HG | Paper | github
- Publisher: Beihang University et al.
- Size: 1317 instances
- License: -
- Question Type: Multi
- Evaluation Method: ME
- Focus: The performance in IT-related tasks
- Numbers of Evaluation Categories/Subcategories: 9/-
- Evaluation Category: Information security, Application, System architecture, Software architecture, Middleware, Network, Operating system, Infrastructure, Database
- Domain: IT
MINT 2023-9 | All | EN | CI | Paper | Github | Dataset | Webサイト
- Publisher: University of Illinois Urbana-Champaign et al.
- Size: 586 instances
- License: Apache-2.0
- Question Type: SQ
- Evaluation Method: CE
- Focus: Solve complex tasks through multi-turn interactions using tools and leveraging natural language feedback
- Numbers of Evaluation Categories/Subcategories: 3/-
- Evaluation Category: Code generation, Decision making, Reasoning
- Domain: Multi-turn interactions
PromptBench 2023-6 | All | EN | CI | Paper | github
- Publisher: Microsoft Research et al.
- Size: 583884 instances
- License: MIT
- Question Type: SQ
- Evaluation Method: CE
- Focus: The models' robustness
- Numbers of Evaluation Categories/Subcategories: 10/15
- Evaluation Category: Sentiment analysis, Grammar correctness, Duplicate sentence detection, Natural language inference, etc.
- Domain: Robustness
EmotionBench 2023-8 | All | EN | HG & MC | Paper | github
- Publisher: The Chinese University of Hong Kong et al.
- サイズ: -
- License: GPL-3.0
- Question Type: SQ
- Evaluation Method: CE
- Focus: The empathy ability
- Numbers of Evaluation Categories/Subcategories: 8/36
- Evaluation Category: Anger, Anxiety, Depression, Frustration, Jealous, Guilt, Fear, Embarrassment
- Domain: Sentiment
Evaluation Platform
CLUE Benchmark Series
- SuperCLUE-Agent
- SuperCLUE-Auto
- SuperCLUE-Math6
- SuperCLUE-Safety
- SuperCLUE-Code3
- SuperCLUE-Video
- SuperCLUE-RAG
- SuperCLUE-Industry
- SuperCLUE-Role
OpenLLM Leaderboard
OpenCompass
MTEB Leaderboard
C-MTEB Leaderboard
Traditional NLP Datasets
Diverging from instruction fine-tuning datasets, we categorize text datasets dedicated to natural language tasks before the widespread adoption of LLMs as traditional NLP datasets.
Dataset information format:
- Dataset name Release Time | Language | Paper | Github | Dataset | Website
- Publisher:
- Train/Dev/Test/All Size:
- License:
- Number of Entity Categories: (NER Task)
- Number of Relationship Categories: (RE Task)
Question Answering
The task of question-answering requires the model to utilize its knowledge and reasoning capabilities to respond to queries based on provided text (which may be optional) and questions.
読解
The task of reading comprehension entails presenting a model with a designated text passage and associated questions, prompting the model to understand the text for the purpose of answering the questions.
Selection & Judgment
BoolQ 2019-5 | EN | Paper | github
- Publisher: University of Washington et al.
- Train/Dev/Test/All Size: 9427/3270/3245/15942
- License: CC-SA-3.0
CosmosQA 2019-9 | EN | Paper | Github | Dataset | Webサイト
- Publisher: University of Illinois Urbana-Champaign et al.
- Train/Dev/Test/All Size: 25588/3000/7000/35588
- License: CC-BY-4.0
CondaQA 2022-11 | EN | Paper | Github | Dataset
- Publisher: Carnegie Mellon University et al.
- Train/Dev/Test/All Size: 5832/1110/7240/14182
- License: Apache-2.0
PubMedQA 2019-9 | EN | Paper | Github | Dataset | Webサイト
- Publisher: University of Pittsburgh et al.
- Train/Dev/Test/All Size: -/-/-/273.5K
- License: MIT
MultiRC 2018-6 | EN | Paper | Github | Dataset
- Publisher: University of Pennsylvania et al.
- Train/Dev/Test/All Size: -/-/-/9872
- License: MultiRC License
RACE 2017-4 | EN | Paper | Dataset | Webサイト
- Publisher: Carnegie Mellon University
- Train/Dev/Test/All Size: 87866/4887/4934/97687
- License: -
C3 2019-4 | ZH | Paper | Github | Webサイト
- Publisher: Cornell University et al.
- Train/Dev/Test/All Size: 11869/3816/3892/19577
- License: -
ReClor 2020-2 | EN | Paper | Webサイト
- Publisher: National University of Singapore
- Train/Dev/Test/All Size: 4638/500/1000/6138
- License: -
DREAM 2020-2 | EN | Paper | Github | Webサイト
- Publisher: National University of Singapore
- Train/Dev/Test/All Size: 4638/500/1000/6138
- License: -
QuAIL 2020-4 | EN | Paper | Webサイト
- Publisher: University of Massachusetts Lowell
- Train/Dev/Test/All Size: 10346/-/2164/12510
- License: CC-NC-SA-4.0
DuReader Yes/No 2019-12 | ZH | Github1 | Github2
- Publisher: Baidu Inc. et al.
- Train/Dev/Test/All Size: 75K/5.5K/11K/91.5K
- License: Apache-2.0
MCTest 2013-10 | EN | Paper | Dataset
- Publisher: Microsoft Research
- Train/Dev/Test/All Size: 1200/200/600/2000
- License: -
Cloze Test
ChID 2019-6 | ZH | Paper | Github | Dataset
- Publisher: Tsinghua University et al.
- Train/Dev/Test/All Size: 605k/23.2K/83.3K/711.5K
- License: Apache-2.0
LAMBADA 2016-6 | EN | Paper | Dataset | Webサイト
- Publisher: University of Trento et al.
- Train/Dev/Test/All Size: 2662/4869/5153/12684
- License: CC-BY-4.0
CLOTH 2018-10 | EN | Paper | Dataset
- Publisher: Carnegie Melon University
- Train/Dev/Test/All Size: 76850/11067/11516/99433
- License: MIT
CMRC2019 2020-12 | ZH | Paper | Github | Webサイト
- Publisher: Harbin Institute of Technology et al.
- Train/Dev/Test/All Size: 100009/3053/5118/108180
- License: CC-BY-SA-4.0
Answer Extraction
SQuAD 2016-11 | EN | Paper | Dataset
- Publisher: Stanford University
- Train/Dev/Test/All Size: 87599/10570/9533/107702
- License: CC-BY-4.0
SQuAD 2.0 2018-6 | EN | Paper | Dataset
- Publisher: Stanford University
- Train/Dev/Test/All Size: 130319/11873/8862/151054
- License: CC-BY-SA-4.0
HOTPOTQA 2018-9 | EN | Paper | Dataset | Webサイト
- Publisher: Carnegie Mellon University et al.
- Train/Dev/Test/All Size: 90447/7405/7405/105257
- License: CC-BY-SA-4.0
TriviaQA 2017-7 | EN | Paper | Github | Dataset
- Publisher: Univ. of Washington et al.
- Train/Dev/Test/All Size: -/-/-/95000
- License: Apache-2.0
Natural Questions 2019-X | EN | Paper | Github | Dataset
- Publisher: Google Research
- Train/Dev/Test/All Size: 307372/7830/7842/323044
- License: CC-BY-4.0
ReCoRD 2018-10 | EN | Paper | Webサイト
- Publisher: Johns Hopkins University et al.
- Train/Dev/Test/All Size: 100730/10000/10000/120730
- License: -
QuAC 2018-8 | EN | Paper | Dataset | Webサイト
- Publisher: AI2 et al.
- Train/Dev/Test/All Size: 83568/7354/7353/98407
- License: CC-BY-SA-4.0
TyDiQA 2020-3 | Multi (11) | Paper | Github | Dataset
- Publisher: Google Research
- Train/Dev/Test/All Size: 116916/18670/18751/154337
- License: Apache-2.0
CMRC2018 2019-11 | ZH | Paper | github
- Publisher: Harbin Institute of Technology et al.
- Train/Dev/Test/All Size: 10321/3351/4895/18567
- License: CC-BY-SA-4.0
Adversarial QA 2020-2 | EN | Paper | Github | Dataset
- Publisher: University College London
- Train/Dev/Test/All Size: 30000/3000/3000/36000
- License: MIT
Quoref 2019-8 | EN | Paper | Webサイト
- Publisher: AI2 et al.
- Train/Dev/Test/All Size: 19399/2418/2537/24354
- License: CC-BY-4.0
MLQA 2020-7 | Multi (7) | Paper | Github | Dataset
- Publisher: Facebook AI Research et al.
- Train/Dev/Test/All Size: -/4199/42246/46445
- License: CC-BY-SA-3.0
DuReader Robust 2020-3 | ZH | Paper | Github1 | Github2
- Publisher: Baidu Inc. et al.
- Train/Dev/Test/All Size: 15K/1.4K/4.8K/21.2K
- License: Apache-2.0
DuReader Checklist 2021-3 | ZH | Github1 | Github2
- Publisher: Baidu Inc. et al.
- Train/Dev/Test/All Size: 3K/1.1K/4.5K/8.6K
- License: Apache-2.0
CUAD 2021-3 | EN | Paper | Dataset
- Publisher: UC Berkeley et al.
- Train/Dev/Test/All Size: 22450/-/4182/26632
- License: CC-BY-4.0
MS MARCO 2016-11 | EN | Paper | Github | Dataset
- Publisher: Microsoft AI & Research
- Train/Dev/Test/All Size: 808731/101093/101092/1010916
- License: MIT
Unrestricted QA
DROP 2019-6 | EN | Paper | Webサイト
- Publisher: University of California et al.
- Train/Dev/Test/All Size: 77409/9536/9622/96567
- License: CC-BY-4.0
CoQA 2018-8 | EN | Paper | Webサイト
- Publisher: Stanford University
- Train/Dev/Test/All Size: -/-/-/127K
- License: -
QASPER 2021-5 | EN | Paper | Webサイト
- Publisher: AI2 et al.
- Train/Dev/Test/All Size: -/-/-/5049
- License: CC-BY-4.0
DuoRC 2018-7 | EN | Paper | Dataset | Webサイト
- Publisher: IBM Research et al.
- Train/Dev/Test/All Size: 130261/27914/27914/186089
- License: MIT
DuReader 2.0 2018-4 | ZH | Paper | Github1 | Github2
- Publisher: Baidu Inc. et al.
- Train/Dev/Test/All Size: -/-/-/200K
- License: Apache-2.0
Knowledge QA
In the knowledge QA task, models respond to questions by leveraging world knowledge, common sense, scientific insights, domain-specific information, and more.
ARC 2018-3 | EN | Paper | Webサイト
- Publisher: AI2
- Train/Dev/Test/All Size: 3370/869/3548/7787
- License: CC-BY-SA
CommonsenseQA 2018-11 | EN | Paper | Github | Dataset | Webサイト
- Publisher: Tel-Aviv University et al.
- Train/Dev/Test/All Size: 9797/1225/1225/12247
- License: MIT
OpenBookQA 2018-10 | EN | Paper | Github | Dataset
- Publisher: AI2 et al.
- Train/Dev/Test/All Size: 4957/500/500/5957
- License: Apache-2.0
PIQA 2019-11 | EN | Paper | Github | Dataset
- Publisher: AI2 et al.
- Train/Dev/Test/All Size: 16.1K/1.84K/3.08K/21.02K
- License: MIT
JEC-QA 2019-11 | EN | Paper | Github | Dataset | Webサイト
- Publisher: Tsinghua University et al.
- Train/Dev/Test/All Size: -/-/26365/26365
- License: CC-NC-ND-4.0
CMD 2019-X | ZH | Github | Dataset
- Publisher: Toyhom
- Train/Dev/Test/All Size: -/-/-/792099
- License: MIT
cMedQA2 2018-11 | ZH | Paper | Dataset
- Publisher: National University of Defense Technology
- Train/Dev/Test/All Size: 100000/4000/4000/108000
- License: GPL-3.0
HEAD-QA 2019-7 | EN & ES | Paper | Github | Dataset | Webサイト
- Publisher: Universidade da Coruna
- Train/Dev/Test/All Size: 2657/1366/2742/13530
- License: MIT
SciQ 2017-9 | EN | Paper | Dataset | Webサイト
- Publisher: University College London et al.
- Train/Dev/Test/All Size: 11679/1000/1000/13679
- License: CC-BY-NC-3.0
WikiQA 2015-9 | EN | Paper | Dataset | Webサイト
- Publisher: Georgia Institute of Technology et al.
- Train/Dev/Test/All Size: 2118/296/633/3047
- License: Microsoft Research Data License
ECQA 2021-8 | EN | Paper | github
- Publisher: IIT Delhi et al.
- Train/Dev/Test/All Size: 7598/1090/2194/10882
- License: CDLA-Sharing-1.0
PsyQA 2021-6 | ZH | Paper | github
- Publisher: The CoAI group et al.
- Train/Dev/Test/All Size: -/-/-/22346
- License: PsyQA User Agreement
WebMedQA 2018-12 | ZH | Paper | github
- Publisher: Chinese Academy of Sciences et al.
- Train/Dev/Test/All Size: 50610/6337/6337/63284
- License: Apache-2.0
WebQuestions 2013-10 | EN | Paper | Dataset
- Publisher: Stanford University
- Train/Dev/Test/All Size: 3778/-/2032/5810
- License: -
Reasoning QA
The focal point of reasoning QA tasks is the requirement for models to apply abilities such as logical reasoning, multi-step inference, and causal reasoning in answering questions.
STRATEGYQA 2021-1 | EN | Paper | Webサイト
- Publisher: Tel Aviv University et al.
- Train/Dev/Test/All Size: 2290/-/490/2780
- License: MIT
COPA 2011-6 | EN | Paper | Webサイト
- Publisher: Indiana University et al.
- Train/Dev/Test/All Size: -/500/500/1000
- License: BSD 2-Clause
HellaSwag 2019-7 | EN | Paper | github
- Publisher: University of Washington et al.
- Train/Dev/Test/All Size: 39905/10042/10003/59950
- License: MIT
StoryCloze 2016-6 | EN | Paper | Dataset
- Publisher: University of Rochester et al.
- Train/Dev/Test/All Size: -/1871/1871/3742
- License: -
Social IQa 2019-4 | EN | Paper | Dataset
- Publisher: AI2
- Train/Dev/Test/All Size: 33410/1954/-/35364
- License: -
LogiQA 2020-7 | EN & ZH | Paper | github
- Publisher: Fudan University et al.
- Train/Dev/Test/All Size: 7376/651/651/8678
- License: -
PROST 2021-8 | EN | Paper | Github | Dataset
- Publisher: University of Colorado Boulder
- Train/Dev/Test/All Size: -/-/18736/18736
- License: Apache-2.0
QuaRTz 2019-11 | EN | Paper | Dataset | Webサイト
- Publisher: AI2
- Train/Dev/Test/All Size: 2696/384/784/3864
- License: CC-BY-4.0
WIQA 2019-9 | EN | Paper | Dataset | Webサイト
- Publisher: AI2
- Train/Dev/Test/All Size: 29808/6894/3993/40695
- License: -
QASC 2019-10 | EN | Paper | Dataset | Webサイト
- Publisher: AI2 et al.
- Train/Dev/Test/All Size: 8134/926/920/9980
- License: CC-BY-4.0
QuaRel 2018-11 | EN | Paper | Webサイト
- Publisher: AI2
- Train/Dev/Test/All Size: 1941/278/552/2771
- License: CC-BY-4.0
ROPES 2019-8 | EN | Paper | Dataset | Webサイト
- Publisher: AI2
- Train/Dev/Test/All Size: 10K/1.6K/1.7K/13.3K
- License: CC-BY-4.0
CREAK 2021-9 | EN | Paper | github
- Publisher: The University of Texas at Austin
- Train/Dev/Test/All Size: 10176/1371/1371/13418
- License: MIT
Recognizing Textual Entailment
The primary objective of tasks related to Recognizing Textual Entailment (RTE) is to assess whether information in one textual segment can be logically inferred from another.
ANLI 2019-10 | EN | Paper | Github | Dataset
- Publisher: UNC Chapel Hill et al.
- Train/Dev/Test/All Size: 162865/3200/3200/169265
- License: CC-NC-4.0
RTE - | EN | Paper1 | Paper2 | Paper3 | Paper4 | Dataset
- Publisher: The PASCAL Recognising Textual Entailment Challenge
- Train/Dev/Test/All Size: 2.49K/277/3K/5.77K
- License: CC-BY-4.0
WANLI 2022-1 | EN | Paper | Dataset
- Publisher: University of Washington et al.
- Train/Dev/Test/All Size: 102885/-/5000/107885
- License: CC-BY-4.0
MedNLI 2018-8 | EN | Paper | Github | Dataset | Webサイト
- Publisher: University of Massachusetts Lowell et al.
- Train/Dev/Test/All Size: 11232/1395/1422/14049
- License: -
CommitmentBank 2019-X | EN | Paper | Github | Dataset
- Publisher: The Ohio State University et al.
- Train/Dev/Test/All Size: -/-/-/1200
- License: -
MultiNLI 2018-6 | EN | Paper | Dataset
- Publisher: New York University
- Train/Dev/Test/All Size: 392702/19647/-/412349
- License: -
SNLI 2015-8 | EN | Paper | Dataset
- Publisher: Stanford Linguistics et al.
- Train/Dev/Test/All Size: 550152/10000/10000/570152
- License: CC-BY-SA-4.0
OCNLI 2020-10 | ZH | Paper | github
- Publisher: Indiana University et al.
- Train/Dev/Test/All Size: 50K/3K/3K/56K
- License: CC-BY-NC-2.0
CMNLI 2020-12 | ZH | Github | Dataset
- Publisher: CLUE team
- Train/Dev/Test/All Size: 391783/12426/13880/418089
- License: -
CINLID 2021-4 | ZH | Dataset
- Publisher: Gao et al.
- Train/Dev/Test/All Size: 80124/-/26708/106832
- License: -
数学
Mathematical assignments commonly involve standard mathematical calculations, theorem validations, and mathematical reasoning tasks, among others.
GSM8K 2021-10 | EN | Paper | Github | Dataset
- Publisher: OpenAI
- Train/Dev/Test/All Size: 7.5K/-/1K/8.5K
- License: MIT
SVAMP 2021-3 | EN | Paper | github
- Publisher: Microsoft Research India
- Train/Dev/Test/All Size: -/-/-/1000
- License: MIT
ASDiv 2021-6 | EN | Paper | Github | Dataset
- Publisher: Institute of Information Science
- Train/Dev/Test/All Size: -/-/-/2305
- License: CC-BY-NC-4.0
MATH 2021-3 | EN | Paper | Github | Dataset
- Publisher: UC Berkeley et al.
- Train/Dev/Test/All Size: 7500/-/5000/12500
- License: MIT
Ape210K 2020-9 | ZH | Paper | github
- Publisher: Yuanfudao AI Lab et al.
- Train/Dev/Test/All Size: 200488/5000/5000/210488
- License: -
Math23K 2017-9 | ZH | Paper | github
- Publisher: Tencent AI Lab
- Train/Dev/Test/All Size: -/-/-/23161
- License: MIT
MathQA 2019-5 | EN | Paper | Dataset | Webサイト
- Publisher: University of Washington et al.
- Train/Dev/Test/All Size: 29837/4475/2985/37297
- License: Apache-2.0
AQUA-RAT 2017-7 | EN | Paper | Github | Dataset
- Publisher: DeepMind
- Train/Dev/Test/All Size: 100949/250/250/101499
- License: Apache-2.0
NaturalProofs 2021-4 | EN | Paper | github
- Publisher: University of Washington et al.
- Train/Dev/Test/All Size: -/-/-/80795
- License: MIT
Coreference Resolution
The core objective of tasks related to coreference resolution is the identification of referential relationships within texts.
WSC 2012-X | EN | Paper | Dataset
- Publisher: University of Toronto et al.
- Train/Dev/Test/All Size: -/-/285/285
- License: CC-BY-4.0
DPR 2012-7 | EN | Paper | Dataset
- Publisher: University of Texas at Dallas
- Train/Dev/Test/All Size: 1322/-/564/1886
- License: -
WinoGrande 2019-7 | EN | Paper | Github | Dataset
- Publisher: AI2 et al.
- Train/Dev/Test/All Size: 63238/1267/1767/66272
- License: CC-BY
WiC 2018-8 | EN | Paper | Webサイト
- Publisher: University of Cambridge
- Train/Dev/Test/All Size: 5428/638/1400/7466
- License: CC-NC-4.0
WinoWhy 2020-7 | EN | Paper | github
- Publisher: HKUST
- Train/Dev/Test/All Size: -/-/-/43972
- License: MIT
CLUEWSC2020 2020-12 | ZH | Paper | Github1 | Github2
- Publisher: CLUE team
- Train/Dev/Test/All Size: 1244/304/290/1838
- License: -
Sentiment Analysis
The sentiment analysis task, commonly known as emotion classification, seeks to analyze and deduce the emotional inclination of provided texts, commonly categorized as positive, negative, or neutral sentiments.
IMDB 2011-6 | EN | Paper | Dataset
- Publisher: Stanford University
- Train/Dev/Test/All Size: 25000/-/25000/50000
- License: -
Sentiment140 2009-X | EN | Paper | Dataset
- Publisher: Stanford University
- Train/Dev/Test/All Size: 1600000/-/359/1600359
- License: -
SST-2 2013-10 | EN | Paper | Dataset
- Publisher: Stanford University
- Train/Dev/Test/All Size: 67349/872/1821/70042
- License: -
EPRSTMT 2021-7 | ZH | Paper | github
- Publisher: CLUE team
- Train/Dev/Test/All Size: 32/32/1363/20992
- License: -
Semantic Matching
The task of semantic matching entails evaluating the semantic similarity or degree of correspondence between two sequences of text.
MRPC 2005-X | EN |紙
- Publisher: Microsoft Research
- Train/Dev/Test/All Size: 4076/-/1725/5801
- License: -
QQP 2018-11 | EN | Paper | Dataset
- Publisher: New York University et al.
- Train/Dev/Test/All Size: 364K/-/-/364K
- License: -
PAWS 2019-6 | EN | Paper | Github | Dataset
- Publisher: Google AI Language
- Train/Dev/Test/All Size: 49401/8000/8000/65401
- License: -
STSB 2017-8 | Multi (10) | Paper | Github | Dataset | Webサイト
- Publisher: Google Research et al.
- Train/Dev/Test/All Size: 5749/1500/1379/8628
- License: -
AFQMC 2020-12 | ZH |紙
- Publisher: CLUE team
- Train/Dev/Test/All Size: 34.3K/4.3K/3.9K/42.5K
- License: -
BQ 2018-10 | ZH | Paper | Dataset
- Publisher: Harbin Institute of Technology et al.
- Train/Dev/Test/All Size: 100000/10000/10000/120000
- License: -
LCQMC 2018-8 | ZH |紙
- Publisher: Harbin Institute of Technology et al.
- Train/Dev/Test/All Size: 238766/8802/12500/260068
- License: CC-BY-4.0
PAWS-X 2019-8 | Multi (6) | Paper | github
- Publisher: Google Research
- Train/Dev/Test/All Size: 296406/11815/11844/320065
- License: -
BUSTM 2021-7 | ZH | Paper | github
- Publisher: CLUE team
- Train/Dev/Test/All Size: 32/32/3772/8087
- License: -
DuQM 2021-9 | ZH | Paper | Github1 | Github2
- Publisher: Baidu Inc. et al.
- Train/Dev/Test/All Size: -/-/-/10121
- License: Apache-2.0
Text Generation
The narrow definition of text generation tasks is bound by provided content and specific requirements. It involves utilizing benchmark data, such as descriptive terms and triplets, to generate corresponding textual descriptions.
CommonGen 2019-11 | EN | Paper | Github | Dataset
- Publisher: University of Southern California et al.
- Train/Dev/Test/All Size: 67389/4018/1497/72904
- License: MIT
DART 2020-7 | EN | Paper | Github | Dataset
- Publisher: Yale University et al.
- Train/Dev/Test/All Size: 30526/2768/6959/40253
- License: MIT
E2E 2017-6 | EN | Paper | Github | Dataset
- Publisher: Heriot-Watt University
- Train/Dev/Test/All Size: 42061/4672/4693/51426
- License: CC-BY-SA-3.0
WebNLG 2017-7 | EN & RU | Paper | Github | Dataset
- Publisher: LORIA et al.
- Train/Dev/Test/All Size: 49665/6490/7930/64085
- License: CC-BY-NC-SA-4.0
テキスト翻訳
Text translation involves transforming text from one language to another.
Text Summarization
The task of text summarization pertains to the extraction or generation of a brief summary or headline from an extended text to encapsulate its primary content.
AESLC 2019-7 | EN | Paper | Github | Dataset
- Publisher: Yale University et al.
- Train/Dev/Test/All Size: 14436/1960/1906/18302
- License: CC-BY-NC-SA-4.0
CNN-DM 2017-4 | EN | Paper | Dataset
- Publisher: Stanford University et al.
- Train/Dev/Test/All Size: 287113/13368/11490/311971
- License: Apache-2.0
MultiNews 2019-7 | EN | Paper | Github | Dataset
- Publisher: Yale University
- Train/Dev/Test/All Size: 44972/5622/5622/56216
- License: -
Newsroom 2018-6 | EN | Paper | Dataset
- Publisher: Cornell University
- Train/Dev/Test/All Size: 995041/108837/108862/1212740
- License: -
SAMSum 2019-11 | EN | Paper | Dataset
- Publisher: Cornell University
- Train/Dev/Test/All Size: 14732/818/819/16369
- License: CC-BY-NC-ND-4.0
XSum 2018-10 | EN | Paper | Github | Dataset
- Publisher: University of Edinburgh
- Train/Dev/Test/All Size: 204045/11332/11334/226711
- License: MIT
Opinion Abstracts 2016-6 | EN | Paper | Dataset
- Publisher: Northeastern University et al.
- Train/Dev/Test/All Size: 5990/-/-/5990
- License: -
WikiLingua 2020-10 | Multi (18) | Paper | Github | Dataset
- Publisher: Columbia University et al.
- Train/Dev/Test/All Size: -/-/-/770087
- License: CC-BY-3.0
LCSTS 2015-6 | ZH | Paper | Dataset
- Publisher: Harbin Institute of Technology
- Train/Dev/Test/All Size: 2400000/10000/1000/2411000
- License: CC-BY-4.0
CNewSum 2021-10 | ZH | Paper | Github | Dataset | Webサイト
- Publisher: ByteDance
- Train/Dev/Test/All Size: 275596/14356/14355/304307
- License: Apache-2.0
XL-Sum 2021-8 | Multi (45) | Paper | Dataset
- Publisher: BUET et al.
- Train/Dev/Test/All Size: 1122857/114198/114198/1351253
- License: CC-BY-NC-SA-4.0
WikiHow 2018-10 | EN | Paper | github
- Publisher: University of California
- Train/Dev/Test/All Size: -/-/-/230K
- License: CC-BY-NC-SA
MediaSum 2021-3 | EN | Paper | Github | Dataset
- Publisher: Microsoft Cognitive Services Research Group
- Train/Dev/Test/All Size: 443596/10000/10000/463596
- License: -
Text Classification
Text classification tasks aim to assign various text instances to predefined categories, comprising text data and category labels as pivotal components.
AGNEWS 2015-9 | EN | Paper | Dataset | Webサイト
- Publisher: New York University
- Train/Dev/Test/All Size: 120000/-/7600/127600
- License: -
TNEWS 2020-11 | ZH | Paper | Github | Dataset
- Publisher: CLUE team
- Train/Dev/Test/All Size: 53.3K/10K/10K/73.3K
- License: -
IFLYTEK 2020-12 | ZH |紙
- Publisher: CLUE team
- Train/Dev/Test/All Size: 12.1K/2.6K/2.6K/17.3K
- License: -
MARC 2020-11 | Multi (6) | Paper | Dataset
- Publisher: Amazon et al.
- Train/Dev/Test/All Size: 1200000/30000/30000/1260000
- License: -
THUCNews 2016-X | ZH | Github | Webサイト
- Publisher: Tsinghua University
- Train/Dev/Test/All Size: -/-/-/1672165
- License: MIT
CSLDCP 2021-7 | ZH | Paper | Github | Webサイト
- Publisher: CLUE team
- Train/Dev/Test/All Size: 536/536/4783/23966
- License: -
Text Quality Evaluation
The task of text quality evaluation, also referred to as text correction, involves the identification and correction of grammatical, spelling, or language usage errors in text.
CoLA 2018-5 | EN | Paper | Webサイト
- Publisher: New York University
- Train/Dev/Test/All Size: 8511/1043/-/9554
- License: CC-BY-4.0
SIGHAN - | ZH | Paper1 | Paper2 | Paper3 | Dataset1 | Dataset2 | Dataset3
- Publisher: Chaoyang Univ. of Technology et al.
- Train/Dev/Test/All Size: 6476/-/3162/9638
- License: -
YACLC 2021-12 | ZH | Paper | github
- Publisher: Beijing Language and Culture University et al.
- Train/Dev/Test/All Size: 8000/1000/1000/10000
- License: -
CSCD-IME 2022-11 | ZH | Paper | github
- Publisher: Tencent Inc
- Train/Dev/Test/All Size: 30000/5000/5000/40000
- License: MIT
Text-to-Code
The Text-to-Code task involves models converting user-provided natural language descriptions into computer-executable code, thereby achieving the desired functionality or operation.
MBPP 2021-8 | EN & PL | Paper | github
- Publisher: Google Research
- Train/Dev/Test/All Size: -/-/974/974
- License: -
DuSQL 2020-11 | ZH & PL | Paper | Dataset
- Publisher: Baidu Inc. et al.
- Train/Dev/Test/All Size: 18602/2039/3156/23797
- License: -
CSpider 2019-11 | ZH & PL | Paper | Github | Webサイト
- Publisher: Westlake University
- Train/Dev/Test/All Size: -/-/-/10181
- License: CC-BY-SA-4.0
Spider 2018-9 | EN & PL | Paper | Github | Webサイト
- Publisher: Yale University
- Train/Dev/Test/All Size: -/-/-/10181
- License: CC-BY-SA-4.0
Named Entity Recognition
The Named Entity Recognition (NER) task aims to discern and categorize named entities within a given text.
WUNT2017 2017-9 | EN | Paper | Dataset
- Publisher: Johns Hopkins University et al.
- Train/Dev/Test/All Size: 3394/1009/1287/5690
- License: CC-BY-4.0
- Number of Entity Categories: 6
Few-NERD 2021-5 | EN | Paper | Github | Dataset | Webサイト
- Publisher: Tsinghua University et al.
- Train/Dev/Test/All Size: -/-/-/188200
- License: CC-BY-SA-4.0
- Number of Entity Categories: 66
CoNLL2003 2003-6 | EN & DE | Paper | Dataset
- Publisher: University of Antwerp
- Train/Dev/Test/All Size: 14041/3250/3453/20744
- License: -
- Number of Entity Categories: 4
OntoNotes 5.0 2013-10 | Multi (3) | Paper | Dataset | Webサイト
- Publisher: Boston Childrens Hospital and Harvard Medical School et al.
- Train/Dev/Test/All Size: 59924/8528/8262/76714
- License: -
- Number of Entity Categories: 18
MSRA 2006-7 | ZH | Paper | Dataset
- Publisher: University of Chicago
- Train/Dev/Test/All Size: 46364/-/4365/50729
- License: CC-BY-4.0
- Number of Entity Categories: 3
Youku NER 2019-6 | ZH | Paper | Github | Dataset
- Publisher: Singapore University of Technology and Design et al.
- Train/Dev/Test/All Size: 8001/1000/1001/10002
- License: -
- Number of Entity Categories: 9
Taobao NER 2019-6 | ZH | Paper | Github | Dataset
- Publisher: Singapore University of Technology and Design et al.
- Train/Dev/Test/All Size: 6000/998/1000/7998
- License: -
- Number of Entity Categories: 9
Weibo NER 2015-9 | ZH | Paper | Github | Dataset
- Publisher: Johns Hopkins University
- Train/Dev/Test/All Size: 1350/269/270/1889
- License: CC-BY-SA-3.0
- Number of Entity Categories: 4
CLUENER 2020-1 | ZH | Paper | Github | Dataset
- Publisher: CLUE Organization
- Train/Dev/Test/All Size: 10748/1343/1345/13436
- License: -
- Number of Entity Categories: 10
Resume 2018-7 | ZH | Paper | github
- Publisher: Singapore University of Technology and Design
- Train/Dev/Test/All Size: 3821/463/477/4761
- License: -
- Number of Entity Categories: 8
Relation Extraction
The endeavor of Relation Extraction (RE) necessitates the identification of connections between entities within textual content. This process typically includes recognizing and labeling pertinent entities, followed by the determination of the specific types of relationships that exist among them.
Dialogue RE 2020-7 | EN & ZH | Paper | Github | Webサイト
- Publisher: Tencent AI Lab et al.
- Train/Dev/Test/All Size: 6100/2034/2034/10168
- License: -
- Number of Relationship Categories: 36
TACRED 2017-9 | EN | Paper | Dataset | Webサイト
- Publisher: Stanford University
- Train/Dev/Test/All Size: 68124/22631/15509/106264
- License: LDC
- Number of Relationship Categories: 42
DocRED 2019-7 | EN | Paper | github
- Publisher: Tsinghua University et al.
- Train/Dev/Test/All Size: 1546589/12332/12842/1571763
- License: MIT
- Number of Relationship Categories: 96
FewRel 2018-10 | EN | Paper1 | Paper2 | Github | Webサイト
- Publisher: Tsinghua University
- Train/Dev/Test/All Size: -/-/-/70000
- License: CC-BY-SA-4.0
- Number of Relationship Categories: 100
Multitask
Multitask datasets hold significance as they can be concurrently utilized for different categories of NLP tasks.
CSL 2022-9 | ZH | Paper | github
- Publisher: School of Information Engineering et al.
- Train/Dev/Test/All Size: -/-/-/396209
- License: Apache-2.0
QED 2021-3 | EN | Paper | github
- Publisher: Stanford University et al.
- Train/Dev/Test/All Size: 7638/1355/-/8993
- License: CC-BY-SA-3.0 & GFDL
METS-CoV 2022-9 | EN | Paper | github
- Publisher: Zhejiang University et al.
- Train/Dev/Test/All Size: -/-/-/-
- License: Apache-2.0
Multi-modal Large Language Models (MLLMs) Datasets
Pre-training Corpora
Documents
Instruction Fine-tuning Datasets
Remote Sensing
- MMRS-1M : Multi-sensor remote sensing instruction dataset
- Paper: EarthGPT: A Universal Multi-modal Large Language Model for Multi-sensor Image Comprehension in Remote Sensing Domain
- Github: https://github.com/wivizhang/EarthGPT
Images + Videos
- VideoChat2-IT : Instruction fine-tuning dataset for images/videos
- Paper: MVBench: A Comprehensive Multi-modal Video Understanding Benchmark
- Dataset: https://huggingface.co/datasets/OpenGVLab/VideoChat2-IT
Visual Document Understanding
- InstructDoc : A dataset for zero-shot generalization of visual document understanding
- Paper: InstructDoc: A Dataset for Zero-Shot Generalization of Visual Document Understanding with Instructions
- Github: https://github.com/nttmdlab-nlp/InstructDoc
- Dataset: https://github.com/nttmdlab-nlp/InstructDoc
一般的な
- ALLaVA-4V Data : The multimodal instruction fine-tuning dataset for the ALLaVA model
- Paper: ALLaVA: Harnessing GPT4V-synthesized Data for A Lite Vision-Language Model
- Github: https://github.com/FreedomIntelligence/ALLaVA
- Dataset: https://huggingface.co/datasets/FreedomIntelligence/ALLaVA-4V
Evaluation Datasets
Video Understanding
- MVBench : A comprehensive multi-modal video understanding benchmark
- Paper: MVBench: A Comprehensive Multi-modal Video Understanding Benchmark
- Github: https://github.com/OpenGVLab/Ask-Anything/tree/main/video_chat2
- Dataset: https://huggingface.co/datasets/OpenGVLab/MVBench
主題
Multitask
- MMT-Bench : A comprehensive multimodal benchmark for evaluating large vision-language models towards multitask AGI
- Paper: MMT-Bench: A Comprehensive Multimodal Benchmark for Evaluating Large Vision-Language Models Towards Multitask AGI
- Github: https://github.com/OpenGVLab/MMT-Bench
- Dataset: https://huggingface.co/datasets/Kaining/MMT-Bench
Long Input
- MM-NIAH : The first benchmark specifically designed to systematically evaluate the capability of existing MLLMs to comprehend long multimodal documents
- Paper: Needle In A Multimodal Haystack
- Github: https://github.com/OpenGVLab/MM-NIAH
- Dataset: https://github.com/OpenGVLab/MM-NIAH
Factuality
- MultiTrust : The first comprehensive and unified benchmark on the trustworthiness of MLLMs across five primary aspects: truthfulness, safety, robustness, fairness, and privacy
- Paper: Benchmarking Trustworthiness of Multimodal Large Language Models: A Comprehensive Study
- Github: https://github.com/thu-ml/MMTrustEval
- Website: https://multi-trust.github.io/#leaderboard
医学
MultiMed : A benchmark designed to evaluate and enable large-scale learning across a wide spectrum of medical modalities and tasks
- Paper: MultiMed: Massively Multimodal and Multitask Medical Understanding
MedTrinity-25M : A large-scale multimodal dataset with multigranular annotations for medicine
- Paper: MedTrinity-25M: A Large-scale Multimodal Dataset with Multigranular Annotations for Medicine
- Github: https://github.com/UCSC-VLAA/MedTrinity-25M
- Dataset: https://huggingface.co/datasets/UCSC-VLAA/MedTrinity-25M
- Website: https://yunfeixie233.github.io/MedTrinity-25M/
Image Understanding
- MMIU : A comprehensive evaluation suite designed to assess LVLMs across a wide range of multi-image tasks
- Paper: MMIU: Multimodal Multi-image Understanding for Evaluating Large Vision-Language Models
- Github: https://github.com/OpenGVLab/MMIU
- Dataset: https://huggingface.co/datasets/FanqingM/MMIU-Benchmark
- Website: https://mmiu-bench.github.io/
Retrieval Augmented Generation (RAG) Datasets
CRUD-RAG : A comprehensive Chinese benchmark for RAG
- Paper: CRUD-RAG: A Comprehensive Chinese Benchmark for Retrieval-Augmented Generation of Large Language Models
- Github: https://github.com/IAAR-Shanghai/CRUD_RAG
- Dataset: https://github.com/IAAR-Shanghai/CRUD_RAG
WikiEval : To do correlation analysis of difference metrics proposed in RAGAS
- Paper: RAGAS: Automated Evaluation of Retrieval Augmented Generation
- Github: https://github.com/explodinggradients/ragas
- Dataset: https://huggingface.co/datasets/explodinggradients/WikiEval
RGB : A benchmark for RAG
- Paper: Benchmarking Large Language Models in Retrieval-Augmented Generation
- Github: https://github.com/chen700564/RGB
- Dataset: https://github.com/chen700564/RGB
RAG-Instruct-Benchmark-Tester : An updated benchmarking test dataset for RAG use cases in the enterprise
- Dataset: https://huggingface.co/datasets/llmware/rag_instruct_benchmark_tester
- Website: https://medium.com/@darrenoberst/how-accurate-is-rag-8f0706281fd9
ARES : An automated evaluation framework for RAG
- Paper: ARES: An Automated Evaluation Framework for Retrieval-Augmented Generation Systems
- Github: https://github.com/stanford-futuredata/ARES
- Dataset: https://github.com/stanford-futuredata/ARES
ALCE : The quality assessment benchmark for context and responses
- Paper: Enabling Large Language Models to Generate Text with Citations
- Github: https://github.com/princeton-nlp/ALCE
- Dataset: https://huggingface.co/datasets/princeton-nlp/ALCE-data
CRAG : A comprehensive RAG benchmark
- Paper: CRAG -- Comprehensive RAG Benchmark
- Website: https://www.aicrowd.com/challenges/meta-comprehensive-rag-benchmark-kdd-cup-2024
RAGEval :A framework for automatically generating evaluation datasets to evaluate the knowledge usage ability of different LLMs in different scenarios
- Paper: RAGEval: Scenario Specific RAG Evaluation Dataset Generation Framework
- Github: https://github.com/OpenBMB/RAGEval
- Dataset: https://github.com/OpenBMB/RAGEval
LFRQA :A dataset of human-written long-form answers for cross-domain evaluation in RAG-QA systems
- Paper: RAG-QA Arena: Evaluating Domain Robustness for Long-form Retrieval Augmented Question Answering
- Github: https://github.com/awslabs/rag-qa-arena
MultiHop-RAG : Benchmarking retrieval-augmented generation for multi-hop queries
- Paper: MultiHop-RAG: Benchmarking Retrieval-Augmented Generation for Multi-Hop Queries
- Github: https://github.com/yixuantt/MultiHop-RAG/
- Dataset: https://huggingface.co/datasets/yixuantt/MultiHopRAG
接触
連絡先:
Lianwen Jin:[email protected]
Yang Liu:[email protected]
Due to our current limited human resources to manage such a vast amount of data resources, we regret that we are unable to include all data resources at this moment. If you find any important data resources that have not yet been included, we warmly invite you to submit relevant papers, data links, and other information to us. We will evaluate them, and if appropriate, we will include the data in the Awesome-LLMs-Datasets and the survey paper . Your assistance and support are greatly appreciated!
引用
If you wish to cite this project, please use the following citation format:
@article{liu2024survey,
title={Datasets for Large Language Models: A Comprehensive Survey},
author={Liu, Yang and Cao, Jiahuan and Liu, Chongyu and Ding, Kai and Jin, Lianwen},
journal={arXiv preprint arXiv:2402.18041},
year={2024}
}