MicroMLP é um perceptron multicamada de micro rede neural artificial (usado principalmente em módulos ESP32 e Pycom)
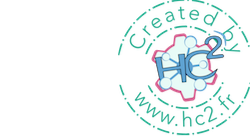
Muito fácil de integrar e muito leve com apenas um arquivo:
Recursos do MicroMLP:
- Estrutura modificável de multicamadas e conexões
- Viés integrado em neurônios
- Plasticidade das conexões incluídas
- Funções de ativação por camada
- Parâmetros Alfa, Eta e Ganho
- Gerenciando conjunto de exemplos e aprendizado
- Funções QLearning para usar aprendizagem por reforço
- Salve e carregue toda a estrutura de/para o arquivo json
- Várias funções de ativação:
- Etapa binária Heaviside
- Logística (sigmóide ou etapa suave)
- Tangente hiperbólica
- Retificador SoftPlus
- ReLU (unidade linear retificada)
- Função gaussiana
Use o aprendizado profundo para:
- Processamento de sinal (processamento de fala, identificação, filtragem)
- Processamento de imagens (compressão, reconhecimento, padrões)
- Controle (diagnóstico, controle de qualidade, robótica)
- Otimização (planejamento, regulação de tráfego, finanças)
- Simulação (simulação de caixa preta)
- Classificação (análise de DNA)
- Aproximação (função desconhecida, função complexa)
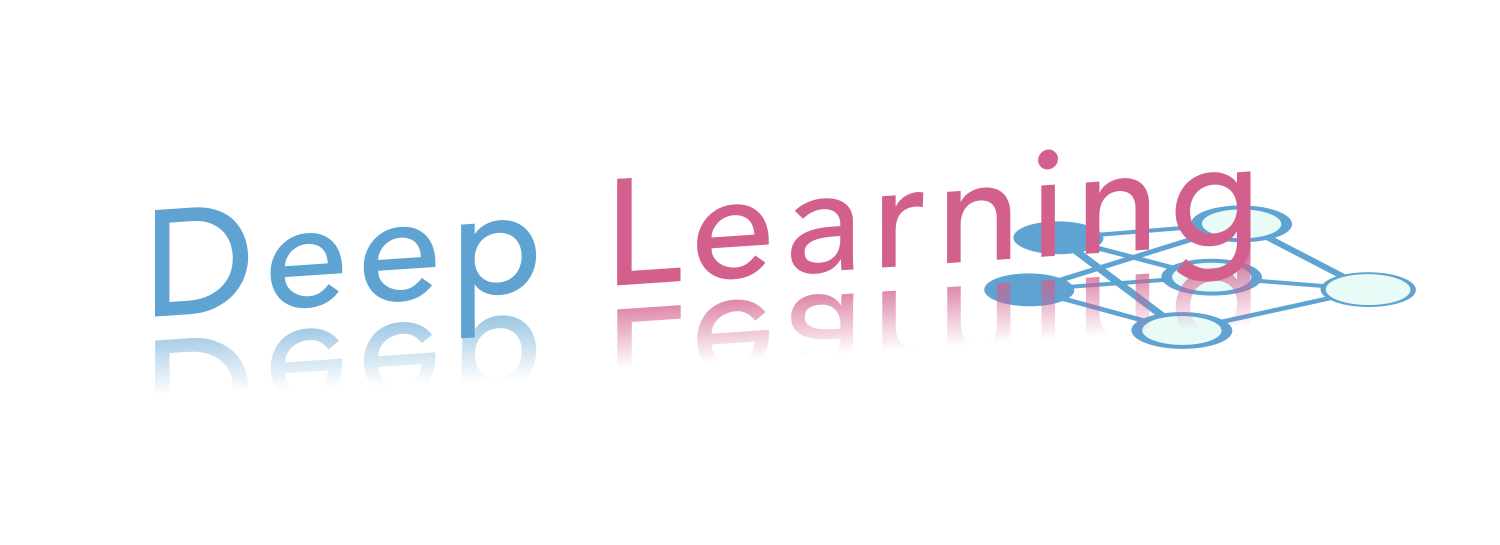
Usando funções estáticas MicroMLP :
Nome | Função |
---|
Criar | mlp = MicroMLP.Create(neuronsByLayers, activationFuncName, layersAutoConnectFunction=None, useBiasValue=1.0) |
CarregarDoArquivo | mlp = MicroMLP.LoadFromFile(filename) |
Usando MicroMLP criação rápida de uma rede neural:
from microMLP import MicroMLP
mlp = MicroMLP . Create ([ 3 , 10 , 2 ], "Sigmoid" , MicroMLP . LayersFullConnect )
Usando a classe principal MicroMLP :
Nome | Função |
---|
Construtor | mlp = MicroMLP() |
Obter camada | layer = mlp.GetLayer(layerIndex) |
ObterLayerIndex | idx = mlp.GetLayerIndex(layer) |
Remover camada | mlp.RemoveLayer(layer) |
ObterInputLayer | inputLayer = mlp.GetInputLayer() |
ObterOutputLayer | outputLayer = mlp.GetOutputLayer() |
Aprender | ok = mlp.Learn(inputVectorNNValues, targetVectorNNValues) |
Teste | ok = mlp.Test(inputVectorNNValues, targetVectorNNValues) |
Prever | outputVectorNNValues = mlp.Predict(inputVectorNNValues) |
QLearningLearnForChosenAction | ok = mlp.QLearningLearnForChosenAction(stateVectorNNValues, rewardNNValue, pastStateVectorNNValues, chosenActionIndex, terminalState=True, discountFactorNNValue=None) |
QLearningPredictBestActionIndex | bestActionIndex = mlp.QLearningPredictBestActionIndex(stateVectorNNValues) |
Salvar em arquivo | ok = mlp.SaveToFile(filename) |
AdicionarExemplo | ok = mlp.AddExample(inputVectorNNValues, targetVectorNNValues) |
Exemplos claros | mlp.ClearExamples() |
AprendaExemplos | learnCount = mlp.LearnExamples(maxSeconds=30, maxCount=None, stopWhenLearned=True, printMAEAverage=True) |
Propriedade | Exemplo | Ler/Escrever |
---|
Camadas | mlp.Layers | pegar |
Contagem de camadas | mlp.LayersCount | pegar |
A rede está completa | mlp.IsNetworkComplete | pegar |
MSE | mlp.MSE | pegar |
MAE | mlp.MAE | pegar |
MSEPercent | mlp.MSEPercent | pegar |
MAEPercent | mlp.MAEPercent | pegar |
Contagem de exemplos | mlp.ExamplesCount | pegar |
Usando MicroMLP para aprender o problema XOr (com tangente hiperbólica):
from microMLP import MicroMLP
mlp = MicroMLP . Create ( neuronsByLayers = [ 2 , 2 , 1 ],
activationFuncName = MicroMLP . ACTFUNC_TANH ,
layersAutoConnectFunction = MicroMLP . LayersFullConnect )
nnFalse = MicroMLP . NNValue . FromBool ( False )
nnTrue = MicroMLP . NNValue . FromBool ( True )
mlp . AddExample ( [ nnFalse , nnFalse ], [ nnFalse ] )
mlp . AddExample ( [ nnFalse , nnTrue ], [ nnTrue ] )
mlp . AddExample ( [ nnTrue , nnTrue ], [ nnFalse ] )
mlp . AddExample ( [ nnTrue , nnFalse ], [ nnTrue ] )
learnCount = mlp . LearnExamples ()
print ( "LEARNED :" )
print ( " - False xor False = %s" % mlp . Predict ([ nnFalse , nnFalse ])[ 0 ]. AsBool )
print ( " - False xor True = %s" % mlp . Predict ([ nnFalse , nnTrue ] )[ 0 ]. AsBool )
print ( " - True xor True = %s" % mlp . Predict ([ nnTrue , nnTrue ] )[ 0 ]. AsBool )
print ( " - True xor False = %s" % mlp . Predict ([ nnTrue , nnFalse ])[ 0 ]. AsBool )
if mlp . SaveToFile ( "mlp.json" ) :
print ( "MicroMLP structure saved!" )
Variável | Descrição | Padrão |
---|
mlp.Eta | Ponderação da correção de erros | 0h30 |
mlp.Alpha | Resistência da plasticidade das conexões | 0,75 |
mlp.Gain | Ganho de aprendizagem em rede | 0,99 |
mlp.CorrectLearnedMAE | Limite de erro de autoaprendizagem | 0,02 |
Gráfico | Nome da função de ativação | Const. | Detalhe |
---|
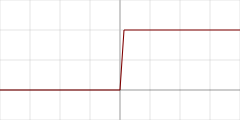 | "Heaviside" | MicroMLP.ACTFUNC_HEAVISIDE | Etapa binária Heaviside |
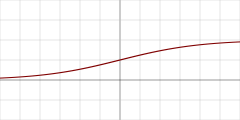 | "Sigmoid" | MicroMLP.ACTFUNC_SIGMOID | Logística (sigmóide ou etapa suave) |
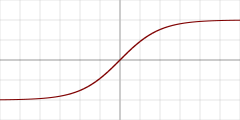 | "TanH" | MicroMLP.ACTFUNC_TANH | Tangente hiperbólica |
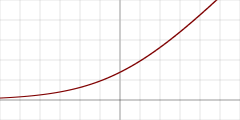 | "SoftPlus" | MicroMLP.ACTFUNC_SOFTPLUS | Retificador SoftPlus |
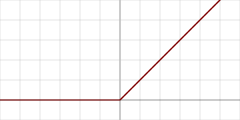 | "ReLU" | MicroMLP.ACTFUNC_RELU | Unidade linear retificada |
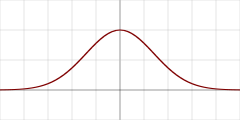 | "Gaussian" | MicroMLP.ACTFUNC_GAUSSIAN | Função gaussiana |
Função de conexão automática de camadas | Detalhe |
---|
MicroMLP.LayersFullConnect | Rede totalmente conectada |
Usando a classe MicroMLP.Layer :
Nome | Função |
---|
Construtor | layer = MicroMLP.Layer(parentMicroMLP, activationFuncName=None, neuronsCount=0) |
ObterLayerIndex | idx = layer.GetLayerIndex() |
ObtenhaNeuron | neuron = layer.GetNeuron(neuronIndex) |
ObterNeuronIndex | idx = layer.GetNeuronIndex(neuron) |
AdicionarNeuron | layer.AddNeuron(neuron) |
Remover Neurônio | layer.RemoveNeuron(neuron) |
GetMeanSquareError | mse = layer.GetMeanSquareError() |
GetMeanAbsoluteError | mae = layer.GetMeanAbsoluteError() |
GetMeanSquareErrorAsPercent | mseP = layer.GetMeanSquareErrorAsPercent() |
GetMeanAbsoluteErrorAsPercent | maeP = layer.GetMeanAbsoluteErrorAsPercent() |
Remover | layer.Remove() |
Propriedade | Exemplo | Ler/Escrever |
---|
ParentMicroMLP | layer.ParentMicroMLP | pegar |
AtivaçãoFuncName | layer.ActivationFuncName | pegar |
Neurônios | layer.Neurons | pegar |
Contagem de neurônios | layer.NeuronsCount | pegar |
Usando a classe MicroMLP.InputLayer(Layer) :
Nome | Função |
---|
Construtor | inputLayer = MicroMLP.InputLayer(parentMicroMLP, neuronsCount=0) |
DefinirInputVectorNNValues | ok = inputLayer.SetInputVectorNNValues(inputVectorNNValues) |
Usando a classe MicroMLP.OutputLayer (Layer) :
Nome | Função |
---|
Construtor | outputLayer = MicroMLP.OutputLayer(parentMicroMLP, activationFuncName, neuronsCount=0) |
GetOutputVectorNNValues | outputVectorNNValues = outputLayer.GetOutputVectorNNValues() |
ComputeTargetLayerError | ok = outputLayer.ComputeTargetLayerError(targetVectorNNValues) |
Usando a classe MicroMLP.Neuron :
Nome | Função |
---|
Construtor | neuron = MicroMLP.Neuron(parentLayer) |
ObterNeuronIndex | idx = neuron.GetNeuronIndex() |
GetInputConnections | connections = neuron.GetInputConnections() |
GetOutputConnections | connections = neuron.GetOutputConnections() |
AdicionarInputConnection | neuron.AddInputConnection(connection) |
AdicionarOutputConnection | neuron.AddOutputConnection(connection) |
RemoverInputConnection | neuron.RemoveInputConnection(connection) |
RemoverOutputConnection | neuron.RemoveOutputConnection(connection) |
Definir Bias | neuron.SetBias(bias) |
GetBias | neuron.GetBias() |
DefinirOutputNNValue | neuron.SetOutputNNValue(nnvalue) |
CalcularValor | neuron.ComputeValue() |
Erro de cálculo | neuron.ComputeError(targetNNValue=None) |
Remover | neuron.Remove() |
Propriedade | Exemplo | Ler/Escrever |
---|
Camada Parental | neuron.ParentLayer | pegar |
Saída computada | neuron.ComputedOutput | pegar |
ComputedDeltaError | neuron.ComputedDeltaError | pegar |
ComputedSignalError | neuron.ComputedSignalError | pegar |
Usando a classe MicroMLP.Connection :
Nome | Função |
---|
Construtor | connection = MicroMLP.Connection(neuronSrc, neuronDst, weight=None) |
AtualizarPeso | connection.UpdateWeight(eta, alpha) |
Remover | connection.Remove() |
Propriedade | Exemplo | Ler/Escrever |
---|
NeuronSrc | connection.NeuronSrc | pegar |
NeuronDst | connection.NeuronDst | pegar |
Peso | connection.Weight | pegar |
Usando a classe MicroMLP.Bias :
Nome | Função |
---|
Construtor | bias = MicroMLP.Bias(neuronDst, value=1.0, weight=None) |
AtualizarPeso | bias.UpdateWeight(eta, alpha) |
Remover | bias.Remove() |
Propriedade | Exemplo | Ler/Escrever |
---|
NeuronDst | bias.NeuronDst | pegar |
Valor | bias.Value | pegar |
Peso | bias.Weight | pegar |
Usando funções estáticas MicroMLP.NNValue :
Nome | Função |
---|
DePorcentagem | nnvalue = MicroMLP.NNValue.FromPercent(value) |
Novo percentual | nnvalue = MicroMLP.NNValue.NewPercent() |
DeByte | nnvalue = MicroMLP.NNValue.FromByte(value) |
NovoByte | nnvalue = MicroMLP.NNValue.NewByte() |
DeBool | nnvalue = MicroMLP.NNValue.FromBool(value) |
NovoBool | nnvalue = MicroMLP.NNValue.NewBool() |
FromAnalogSignal | nnvalue = MicroMLP.NNValue.FromAnalogSignal(value) |
Novo sinal analógico | nnvalue = MicroMLP.NNValue.NewAnalogSignal() |
Usando a classe MicroMLP.NNValue :
Nome | Função |
---|
Construtor | nnvalue = MicroMLP.NNValue(minValue, maxValue, value) |
Propriedade | Exemplo | Ler/Escrever |
---|
AsFloat | nnvalue.AsFloat = 639.513 | obter / definir |
AsInt | nnvalue.AsInt = 12345 | obter / definir |
AsPercent | nnvalue.AsPercent = 65 | obter / definir |
AsByte | nnvalue.AsByte = b'x75' | obter / definir |
AsBool | nnvalue.AsBool = True | obter / definir |
AsAnalogSignal | nnvalue.AsAnalogSignal = 0.39472 | obter / definir |
Por JC`zic para HC² ;')
Mantenha as coisas simples, estúpido ?